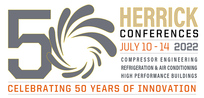
Étude comparative entre la régulation CVC à haute performance basée sur des règles et la régulation fondée sur l'apprentissage par renforcement approfondi dans un système multizone à volume d'air variable.
Comparison study of high-performance rule-based HVAC control with deep reinforcement learning-based control in a multi-zone VAV system.
Résumé
The design, commissioning, and retrofit of heating, ventilation, and air-conditioning (HVAC) control systems are crucially important for energy efficiency but often neglected. Generally, designers and control contractors adopt ad-hoc control sequences based on diffused and fragmented information and therefore the majority of the existing control sequences are diverse and sub-optimal. ASHRAE Guideline 36 (GDL36), High-performance Sequences of Operation for HVAC Systems, is thus developed to provide standardized and high-performance rule-based HVAC control sequences with the main focus on maximizing energy efficiency. However, these high-performance rules-based control sequences are still under-development, and only a few studies verify their overall effectiveness. In addition, the performance evaluations in most existing studies only focus on the energy-saving potentials compared with the conventional rule-based control strategies. In this study, the high-performance rule-based control sequences from GDL36 was compared to the state-of-the-art deep reinforcement (DRL) control in terms of the energy efficiency in a multi-zone VAV system. The system-level supervisory controls (i.e., supply air temperature and supply differential pressure setpoint) in ASHRAE GDL36 were replaced by the counterpart in the DRL control, of which action space is a bi-dimensional continuous space. A five-zone medium office building model in Modelica was utilized as a virtual testbed. Particularly, the plant side power consumption uses a regression model to reflect the real condition of the plant loop operation, Proximal policy optimization (PPO) was selected as the DRL algorithm due to its stable performance for the continuous space and easiness of the hyper-parameter tuning. The DRL algorithm was implemented using the Tianshou library in Python. A containerized OpenAI gym environment was leveraged to enable the connection between the Modelica building model and the DRL algorithm. Typical load conditions in Chicago, 5A (high and mild load weeklong simulation) were considered. The simulation results show that control sequences from GDL36 perform comparable performance in terms of energy efficiency and thermal comfort as the DRL controls.
Documents disponibles
Format PDF
Pages : 10 p.
Disponible
Gratuit
Détails
- Titre original : Comparison study of high-performance rule-based HVAC control with deep reinforcement learning-based control in a multi-zone VAV system.
- Identifiant de la fiche : 30030228
- Langues : Anglais
- Source : 2022 Purdue Conferences. 7th International High Performance Buildings Conference at Purdue.
- Date d'édition : 2022
Liens
Voir d'autres communications du même compte rendu (39)
Voir le compte rendu de la conférence
Indexation
-
Towards higher energy efficiency of residential...
- Auteurs : FREI B., HUBER H.
- Date : 16/06/2013
- Langues : Anglais
- Source : Clima 2013. 11th REHVA World Congress and 8th International Conference on Indoor Air Quality, Ventilation and Energy Conservation in Buildings.
- Formats : PDF
Voir la fiche
-
HVAC industry's mission for sustainability and ...
- Auteurs : SIMHA R. V.
- Date : 01/2008
- Langues : Anglais
- Source : Air Conditioning and Refrigeration Journal - vol. 11 - n. 1
- Formats : PDF
Voir la fiche
-
Building efficiency: a cross-section of comfort...
- Auteurs : DOVJAK M., SHUKUYA M., KRAINER A.
- Date : 16/06/2013
- Langues : Anglais
- Source : Clima 2013. 11th REHVA World Congress and 8th International Conference on Indoor Air Quality, Ventilation and Energy Conservation in Buildings.
- Formats : PDF
Voir la fiche
-
Building case study refurbishment: height of su...
- Auteurs : PEARSON A.
- Date : 11/2011
- Langues : Anglais
- Source : CIBSE Journal - vol. 3 - n. 11
Voir la fiche
-
The application of infrared thermography for th...
- Auteurs : HABAIBEH A. al-, LUKE SIENA F.
- Date : 10/09/2012
- Langues : Anglais
- Source : 4th Jordanian IIR International Conference on Refrigeration and Air Conditioning. Proceedings: Amman, Jordan, September 10-12, 2012.
- Formats : PDF
Voir la fiche