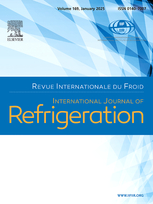
Document IIF
Optimisation bayésienne à dimension réduite pour l’étalonnage de modèles de cycles de compression de vapeur transitoires.
Reduced-dimension Bayesian optimization for model calibration of transient vapor compression cycles.
Auteurs : MA J., KIM D., BRAUN J. E.
Type d'article : Article de la RIF
Résumé
Development and calibration of first-principles dynamic models of vapor compression cycles (VCCs) is of critical importance for applications that include control design and fault detection and diagnostics. Nevertheless, the inherent complexity of models that are represented by large systems of differential–algebraic equations leads to significant challenges for model calibration processes that utilize classical gradient-based methods. Bayesian optimization (BO) is a sample-efficient and gradient-free approach using a probabilistic surrogate model and optimal search over a feasible parameter space. Despite the benefits of BO in reducing computational costs, challenges remain in dealing with a high-dimensional calibration task resulting from a large set of parameters that have significant impacts on system behavior and need to be calibrated simultaneously. This paper presents a reduced-dimension BO framework for calibrating transient VCCs models where the calibration space is projected to a low-dimensional subspace for accelerating convergence of the solution algorithm and consequently reducing the number of transient simulations. The proposed approach was demonstrated via two case studies associated with different VCC applications where 10 parameters were calibrated in each case using laboratory measurements. The reduced-dimension BO framework only required of the iterations associated with a standard BO method that deals with high-dimensional calibration parameters for converged solutions and yielded comparable accuracy. Furthermore, both calibrated models revealed significant accuracy improvements compared to uncalibrated models.
Documents disponibles
Format PDF
Pages : 13
Disponible
Prix public
20 €
Prix membre*
Gratuit
* meilleur tarif applicable selon le type d'adhésion (voir le détail des avantages des adhésions individuelles et collectives)
Détails
- Titre original : Reduced-dimension Bayesian optimization for model calibration of transient vapor compression cycles.
- Identifiant de la fiche : 30032857
- Langues : Anglais
- Source : International Journal of Refrigeration - Revue Internationale du Froid - vol. 168
- Date d'édition : 12/2024
- DOI : http://dx.doi.org/10.1016/j.ijrefrig.2024.09.010
Liens
Voir d'autres articles du même numéro (63)
Voir la source
Indexation
- Thèmes : Systèmes à compression
- Mots-clés : Optimisation; Cycle; Compression mécanique de vapeur; Modélisation
-
Decentralized optimization for vapor compressio...
- Auteurs : ZHAO L., CAI W. J., DING X. D., et al.
- Date : 03/2013
- Langues : Anglais
- Source : Applied Thermal Engineering - vol. 51 - n. 1-2
Voir la fiche
-
Analysis of refrigerating cycles working with i...
- Auteurs : ABLANQUE N., RIGOLA J., OLIET C., et al.
- Date : 25/06/2012
- Langues : Anglais
- Source : 10th IIR-Gustav Lorentzen Conference on Natural Working Fluids (GL2012). Proceedings. Delft, The Netherlands, June 25-27, 2012.
- Formats : PDF
Voir la fiche
-
A review for numerical simulation of vapor comp...
- Auteurs : QIAO H., RADERMACHER R., AUTE V.
- Date : 12/07/2010
- Langues : Anglais
- Source : 2010 Purdue Conferences. 13th International Refrigeration and Air-Conditioning Conference at Purdue.
- Formats : PDF
Voir la fiche
-
Optimal subcooling in vapor compression systems...
- Auteurs : KOELN J., ALLEYNE A. G.
- Date : 07/2014
- Langues : Anglais
- Source : International Journal of Refrigeration - Revue Internationale du Froid - vol. 43
- Formats : PDF
Voir la fiche
-
High-pressure liquid refrigerant injection for ...
- Auteurs : SCHMITT J., LANGEBACH R.
- Date : 08/2024
- Langues : Anglais
- Source : International Journal of Refrigeration - Revue Internationale du Froid - vol. 164
- Formats : PDF
Voir la fiche