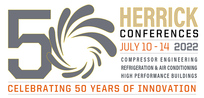
Résumé
Accurately predicting the performance of radiant slab systems can be challenging due to the large thermal capacitance of the radiant slab and room temperature stratification. Current methods for predicting heating and cooling energy consumption of hydronic radiant slabs include detailed first-principles (e.g., finite difference) and reduced-order (e.g., thermal Resistor-Capacitor (RC) network) models. Creating and calibrating detailed first-principles models, as well as detailed RC network models for predicting the performance of radiant slabs require substantial effort. To develop improved control, monitoring, and diagnostic methods, there is a need for simpler models that can be readily trained using in-situ measurements.
In this study, we explored a novel hybrid modeling method that integrates a simple RC network model with an evolving learning-based algorithm termed the Growing Gaussian Mixture Regression (GGMR) modeling approach to predict the heating and cooling rates of a radiant slab system for a Living Laboratory office space. The RC network model predicts heating or cooling load of the radiant slab system that is provided as an input to the GGMR model. Three modeling approaches were considered in this study: 1) an RC network model; 2) a GGMR model, and 3) the proposed hybrid modeling between RC and GGMR. The three modeling methods have been compared for predicting the energy use of a radiant slab system of a Living Laboratory office space using measurement data from January 15th to March 7th, 2022. The first two weeks of data were used for training, while the remaining data was used for testing of all three modeling methods. The hybrid approach had a Normalized Root Mean Square Error (NRMSE) of 15.46 percent (8.62 percent less than the RC-Model 3 alone and 19.36 percent less than the GGMR alone), a Coefficient of Variation of RMSE (CVRMSE) of 6.43 percent (3.59 percent less than the RC-Model 3 and 8.05 percent less than the GGMR), a Mean Absolute Error (MAE) of 3.61 kW (2.13 kW and 3.87 kW less than the RC-Model 3 and GGMR, respectively), and a Mean Absolute Percentage Error (MAPE) of 5.28 percent (3.85 percent and 3.92 percent lower than the RC-Model 3 and GGMR, respectively).
Documents disponibles
Format PDF
Pages : 10 p.
Disponible
Gratuit
Détails
- Titre original : A novel hybrid modeling method for predicting energy use of hydronic radiant.
- Identifiant de la fiche : 30030238
- Langues : Anglais
- Source : 2022 Purdue Conferences. 7th International High Performance Buildings Conference at Purdue.
- Date d'édition : 2022
Liens
Voir d'autres communications du même compte rendu (39)
Voir le compte rendu de la conférence
Indexation
- Thèmes : Conditionnement d'air pour le confort
- Mots-clés : Froid radiatif; Rayonnement; Chauffage; Performance; Modélisation; Prévision
-
Comparison of three transient models for heatin...
- Auteurs : REY A., DUMOULIN R., ATHIENITIS A. K.
- Date : 09/07/2018
- Langues : Anglais
- Source : 2018 Purdue Conferences. 5th International High Performance Buildings Conference at Purdue.
- Formats : PDF
Voir la fiche
-
Anticipating an efficient relative humidity in ...
- Auteurs : NAJAFI N. Z., HAGHIGHI A. P.
- Date : 02/2019
- Langues : Anglais
- Source : International Journal of Refrigeration - Revue Internationale du Froid - vol. 98
- Formats : PDF
Voir la fiche
-
Walls to heat, cool.
- Auteurs : HOLMES H. D., MACPHERSON M. D.
- Date : 09/2011
- Langues : Anglais
- Source : EcoLibrium - vol. 10 - n. 8
- Formats : PDF
Voir la fiche
-
Etude expérimentale sur un plafond rayonnant.
- Auteurs : DI H., WANG W., GE Q., et al.
- Date : 08/2000
- Langues : Chinois
- Source : HV & AC - vol. 30 - n. 135
Voir la fiche
-
Estudio de un sistema de climatización radiante...
- Auteurs : MOIÁ-POL A., ROSSELLÓ BATLE B., MAS GARCIA B.
- Date : 03/05/2016
- Langues : Espagnol
- Source : CYTEF 2016. VIII Congreso Ibérico y VI Congreso Iberoamericano de las Ciencias y Técnicas del Frío, Coimbra-Portugal, 3-6 mayo, 2016.
- Formats : PDF
Voir la fiche