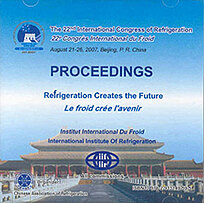
Résumé
Accurate prediction of frost growth is rather difficult because of its typically strong nonlinear and time-dependent process, and the measured experimental data usually contain many noisy signals. To solve this problem, a novel machine learning method-support vector machine (SVM) based on structure risk minimization principle is introduced to develop models for the prediction, during the frost growth, of frost thickness, total heat flux and frost mass concentration. The predicted results are found to be in good agreement with the measured experimental data, with mean relative error less than 0.62% for the total heat flux, 2.42% for the frost mass concentration, and 5.94% for the frost thickness. Compared with the multivariate nonlinear regression model, the SVM models show better capability in solving nonlinear, time-dependent and noise-signal-interfered problem. This demonstrates that the SVM technique can be well used in predicting the frost growth characteristics, and accordingly, help optimize air-to-refrigerant system.
Documents disponibles
Format PDF
Pages : ICR07-B2-185
Disponible
Prix public
20 €
Prix membre*
Gratuit
* meilleur tarif applicable selon le type d'adhésion (voir le détail des avantages des adhésions individuelles et collectives)
Détails
- Titre original : Application of support vector machine for predicting the frost growth on cold surface.
- Identifiant de la fiche : 2008-0220
- Langues : Anglais
- Source : ICR 2007. Refrigeration Creates the Future. Proceedings of the 22nd IIR International Congress of Refrigeration.
- Date d'édition : 21/08/2007
Liens
Voir d'autres communications du même compte rendu (839)
Voir le compte rendu de la conférence
-
ETUDE DES CARACTERISTIQUES ENERGETIQUES DES REF...
- Auteurs : NIKUL'SINA D. G.
- Date : 1984
- Langues : Russe
- Source : Kholodilnaya Tekhnika - n. 10
Voir la fiche
-
Study of frost properties in a low temperature ...
- Auteurs : YAMASHITA K., HAMADA M., ISE S., et al.
- Date : 21/08/2007
- Langues : Anglais
- Source : ICR 2007. Refrigeration Creates the Future. Proceedings of the 22nd IIR International Congress of Refrigeration.
- Formats : PDF
Voir la fiche
-
Transient two-dimensional model of frost format...
- Auteurs : LENIC K., TRP A., FRANKOVIC B.
- Date : 01/2009
- Langues : Anglais
- Source : International Journal of Heat and Mass Transfer - vol. 52 - n. 1-2
Voir la fiche
-
Accroissement de la couche de givre sur les sur...
- Auteurs : BETTANINI E.
- Date : 03/1995
- Langues : Italien
- Source : Industria italiana del Freddo - vol. 39 - n. 2
Voir la fiche
-
Etude expérimentale sur deux types de formation...
- Auteurs : XU X., QU K., DUAN Y.
- Date : 2002
- Langues : Chinois
- Source : Journal of Refrigeration - n. 4
- Formats : PDF
Voir la fiche