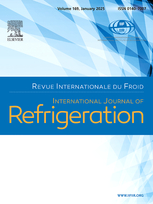
Document IIF
Détection quantitative basée sur des algorithmes d’apprentissage automatique des défauts de charge en frigorigène dans les systèmes de conditionnement d’air à plusieurs unités.
Quantitative detection of refrigerant charge faults in multi-unit air conditioning systems based on machine learning algorithms.
Résumé
Refrigerant charging discrepancies constitute the predominant malfunctions in air conditioning systems. Achieving the optimal charging level is crucial for system performance, underscoring the importance of precise refrigerant level prediction. This study introduces an algorithm designed for the quantitative detection of refrigerant charging errors by integrating the Markov Transition Field (MTF), Convolutional Neural Networks (CNN), and Multi-head Self-Attention (MSA) mechanisms. A high-precision enthalpy difference chamber was employed to establish a Variable Refrigerant Flow (VRF) refrigerant charging test bench. This setup facilitated the analysis of system parameter sensitivity to charging faults and aided in the creation of a training dataset for the algorithm. Comparative analysis was conducted against Support Vector Machines (SVM), Random Forests (RF), CNN with Self-Attention (AT), and MTF-CNN-MSA. The findings reveal that our method adeptly captures temporal dependencies and dynamic shifts in time series as visual representations, offering novel insights for discerning fault patterns within such data. Notably, the maximum pressure variations at high-pressure and low-pressure points were 0.25 MPa and 0.07 MPa, respectively, with temperature shifts of 12 °C and 3.5 °C at the high and low-temperature points. The high-pressure and high-temperature points are particularly sensitive to changes in refrigerant charging, and parameters from these sections were utilized to construct the dataset. The CNN-MSA algorithm demonstrates consistent performance across various fault types, effectively delineating fault characteristics. The accuracies achieved by SVM, RF, CNN-AT, and MTF-CNN-MSA were 84.38 %, 73.75 %, 88.13 %, and 93.75 %, respectively. In comparison, the CNN-MSA algorithm was able to more accurately detect refrigerant charge faults at different levels.
Documents disponibles
Format PDF
Pages : 184-193
Disponible
Prix public
20 €
Prix membre*
Gratuit
* meilleur tarif applicable selon le type d'adhésion (voir le détail des avantages des adhésions individuelles et collectives)
Détails
- Titre original : Quantitative detection of refrigerant charge faults in multi-unit air conditioning systems based on machine learning algorithms.
- Identifiant de la fiche : 30033217
- Langues : Anglais
- Source : International Journal of Refrigeration - Revue Internationale du Froid - vol. 169
- Date d'édition : 01/2025
- DOI : http://dx.doi.org/10.1016/j.ijrefrig.2024.10.026
Liens
Voir d'autres articles du même numéro (33)
Voir la source
-
A variable refrigerant flow (VRF) air-condition...
- Auteurs : CHENG H., MU W., CHENG Y., CHEN H., XING L.
- Date : 21/08/2023
- Langues : Anglais
- Source : Proceedings of the 26th IIR International Congress of Refrigeration: Paris , France, August 21-25, 2023.
- Formats : PDF
Voir la fiche
-
Air-air split AC with minimum charge of propane.
- Auteurs : ANDERSON K., LUNDAHL G., GRANRYD E.
- Date : 13/06/2022
- Langues : Anglais
- Source : 15th IIR-Gustav Lorentzen Conference on Natural Refrigerants (GL2022). Proceedings. Trondheim, Norway, June 13-15th 2022.
- Formats : PDF
Voir la fiche
-
R290 leakage hazards assessment of a 1 HP split...
- Auteurs : NING Q., HE G., SUN W., FAN M., LI X., HONG Z.
- Date : 07/2022
- Langues : Anglais
- Source : International Journal of Refrigeration - Revue Internationale du Froid - vol. 139
- Formats : PDF
Voir la fiche
-
Use of peripheral fins for R-290 charge reducti...
- Auteurs : RIBEIRO G. B., BARBOSA J. Jr
- Date : 10/2019
- Langues : Anglais
- Source : International Journal of Refrigeration - Revue Internationale du Froid - vol. 106
- Formats : PDF
Voir la fiche
-
Fault diagnosis of refrigerant charge based on ...
- Auteurs : LIU J., CHEN H., WANG J., et al.
- Date : 11/07/2016
- Langues : Anglais
- Source : 2016 Purdue Conferences. 16th International Refrigeration and Air-Conditioning Conference at Purdue.
- Formats : PDF
Voir la fiche