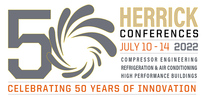
Une approche par réseau neuronal pour développer des corrélations algébriques pour le transfert de chaleur et l'écoulement des fluides.
A neural-network approach to develop algebraic correlations for heat transfer and fluid flow.
Numéro : 2120
Auteurs : LIN L., GAO L., HWANG Y., KEDZIERSKI M.
Résumé
Many heat transfer and fluid flow problems are too complex to model using traditional regression methods. Machine learning (ML) offers a new way to develop predictive models with high accuracy. However, current ML models are often uninterpretable and used as “black boxes”. This paper presents an approach to develop explicit, algebraic correlations from neural networks. An interpretable neural network, namely DimNet, is designed. One can train DimNet with experimental or simulation data and then convert the trained network to an explicit, power-law-like piecewise function. Besides being interpretable, DimNet inherits advantages of neural networks in modeling complex nonlinear problems. The mechanism and effectiveness of DimNet and the correlation development approach is further demonstrated by two case studies: 1) correlating simulation data for the friction factor of flow in smooth pipes; 2) correlating experimental data for flow boiling heat transfer coefficient within microfin tubes. Both case studies show DimNet can produce simple, explicit, algebraic correlations that are both statistically and phenomenologically accurate. The presented approach can be potentially used to develop correlations for various thermal-hydraulic problems, such as the pressure drop and heat transfer of single- and multi-phase flow, heat exchangers, and other thermal-hydraulic equipment.
Documents disponibles
Format PDF
Pages : 10 p.
Disponible
Gratuit
Détails
- Titre original : A neural-network approach to develop algebraic correlations for heat transfer and fluid flow.
- Identifiant de la fiche : 30030473
- Langues : Anglais
- Sujet : Technologie
- Source : 2022 Purdue Conferences. 19th International Refrigeration and Air-Conditioning Conference at Purdue.
- Date d'édition : 10/07/2022
Liens
Voir d'autres communications du même compte rendu (224)
Voir le compte rendu de la conférence
Indexation
-
Prediction of normal boiling point and critical...
- Auteurs : WANG G., HU P.
- Date : 07/2023
- Langues : Anglais
- Source : International Journal of Refrigeration - Revue Internationale du Froid - vol. 151
- Formats : PDF
Voir la fiche
-
Rapid prediction of regenerator performance for...
- Auteurs : CHEN X., LI S., YU J., YANG S., CHEN H.
- Date : 02/2024
- Langues : Anglais
- Source : International Journal of Refrigeration - Revue Internationale du Froid - vol. 158
- Formats : PDF
Voir la fiche
-
Using Deep Learning in Real-Time for Clothing C...
- Auteurs : MEDINA A., MÉNDEZ J. I., PONCE P., PEFFER T., MEIER A., MOLINA A.
- Date : 03/2022
- Langues : Anglais
- Source : Energies - vol. 15 - n. 5
- Formats : PDF
Voir la fiche
-
Evaluating the generality of machine learning-b...
- Auteurs : SHOUREHDELI S. A., GHOLIPOUR H.
- Date : 03/2024
- Langues : Anglais
- Source : International Journal of Refrigeration - Revue Internationale du Froid - vol. 159
- Formats : PDF
Voir la fiche
-
A comparative study of various machine learning...
- Auteurs : BEHNAM P., FAEGH M., SHAFII M. B., KHIADANI M.
- Date : 06/2021
- Langues : Anglais
- Source : International Journal of Refrigeration - Revue Internationale du Froid - vol. 126
- Formats : PDF
Voir la fiche