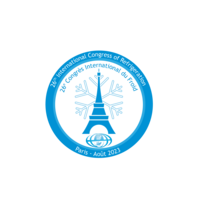
Document IIF
Une méthode d’apprentissage automatique interprétable pour le diagnostic des défaillances des systèmes de chauffage, de ventilation et de conditionnement d’air.
An interpretable machine learning method for fault diagnosis of heating, ventilation and air conditioning systems.
Résumé
Due to the factors such as equipment fault, component wear, and unplanned maintenance, HVAC systems often operate at low energy efficiency, increasing energy consumption, failing to control temperature and humidity, and even causing equipment component damage. Therefore, it is meaningful to study the fault diagnosis for HVAC systems. However, most current machine learning methods are black-box models and extremely hard to interpret or explain, although they have a good performance in fault diagnosis. To fill the gap of poor interpretability of the machine learning algorithm used in HVAC fault diagnosis, this study proposes a novel method based on SHAP (SHapley Additive exPlanation) value, which can visualize the fault diagnosis criteria and show the impact of input variables on the fault diagnostic results, to explain the machine learning method. The proposed method has been verified on the actual chiller and can achieve high diagnostic accuracy for several faults.
Documents disponibles
Format PDF
Pages : 9
Disponible
Prix public
20 €
Prix membre*
Gratuit
* meilleur tarif applicable selon le type d'adhésion (voir le détail des avantages des adhésions individuelles et collectives)
Détails
- Titre original : An interpretable machine learning method for fault diagnosis of heating, ventilation and air conditioning systems.
- Identifiant de la fiche : 30031861
- Langues : Anglais
- Sujet : Technologie
- Source : Proceedings of the 26th IIR International Congress of Refrigeration: Paris , France, August 21-25, 2023.
- Date d'édition : 21/08/2023
- DOI : http://dx.doi.org/10.18462/iir.icr.2023.0501
Liens
Voir d'autres communications du même compte rendu (492)
Voir le compte rendu de la conférence
Indexation
-
A robust fault diagnosis method for HVAC system...
- Auteurs : ZHU X., CHEN S., CHEN K., LIANG X., REN T., JIN X., DU Z.
- Date : 21/08/2023
- Langues : Anglais
- Source : Proceedings of the 26th IIR International Congress of Refrigeration: Paris , France, August 21-25, 2023.
- Formats : PDF
Voir la fiche
-
Integration of dynamic model and classification...
- Auteurs : AGUILERA J. J., MEESENBURG W., SCHULTE A., OMMEN T., MARKUSSEN W. B., ZÜHLSDORF B., POULSEN J. L., FÖRSTERLING S., ELMEGAARD B.
- Date : 13/06/2022
- Langues : Anglais
- Source : 15th IIR-Gustav Lorentzen Conference on Natural Refrigerants (GL2022). Proceedings. Trondheim, Norway, June 13-15th 2022.
- Formats : PDF
Voir la fiche
-
An intelligent fault detection and diagnosis mo...
- Auteurs : WANG Z. W., WANG S. C., LI D., CAO Z. W., HE Y. L.
- Date : 04/2024
- Langues : Anglais
- Source : International Journal of Refrigeration - Revue Internationale du Froid - vol. 160
- Formats : PDF
Voir la fiche
-
A semi-supervised data-driven approach for chil...
- Auteurs : FENG Z., WANG L., MA X., JIANG Z., CHANG B.
- Date : 05/04/2023
- Langues : Anglais
- Source : 3rd IIR conference on HFO Refrigerants and low GWP Blends. Shanghai, China.
- Formats : PDF
Voir la fiche
-
A comprehensive review: Fault detection, diagno...
- Auteurs : SINGH V., MATHUR J., BHATIA A.
- Date : 12/2022
- Langues : Anglais
- Source : International Journal of Refrigeration - Revue Internationale du Froid - vol. 144
- Formats : PDF
Voir la fiche