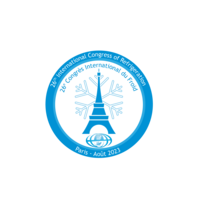
Recommandé par l'IIF / Document IIF
Une méthode robuste de diagnostic des défaillances pour les systèmes de CVC à l’aide d’un apprentissage automatique renforcé par la connaissance du domaine.
A robust fault diagnosis method for HVAC systems with domain knowledge augmented machine learning.
Résumé
The faulty operation of HVAC systems may bring continuous energy waste and end-use energy carbon emissions. Automatic fault diagnosis is an important prerequisite for achieving efficient and smart refrigeration and air conditioning. Data-driven fault diagnosis method with the ability to learn big data has gradually gained widespread attention from academia and industry. However, most of the existing research focus on improving the recognition accuracy for training conditions, while ignoring the generalization for non-training conditions, making it difficult to apply in the scenarios of variable operating conditions that may occur in practical applications. This paper presents a robust fault diagnosis method for HVAC systems by integrating domain knowledge into machine learning. An unsupervised density-based spatial clustering is employed to recognize operating pattern. Deep belief network is used to estimate the fault-free values of fault indicators constructed from expert knowledge. The directional information of performance indexes is learned and integrated with extreme gradient boosting. Various fault simulation and validation experiments are carried out on a real screw chiller. Results reveal that the proposed method yields a significant performance advantage than five traditional data-driven models, especially for non-training conditions, the highest and average improvements are 38.7% and 25.9%, respectively. This research provides a robust and feasible method to guide the on-site application of fault diagnosis for smart HVAC systems.
Documents disponibles
Format PDF
Pages : 8
Disponible
Prix public
20 €
Prix membre*
Gratuit
* meilleur tarif applicable selon le type d'adhésion (voir le détail des avantages des adhésions individuelles et collectives)
Détails
- Titre original : A robust fault diagnosis method for HVAC systems with domain knowledge augmented machine learning.
- Identifiant de la fiche : 30031862
- Langues : Anglais
- Sujet : Technologie
- Source : Proceedings of the 26th IIR International Congress of Refrigeration: Paris , France, August 21-25, 2023.
- Date d'édition : 21/08/2023
- DOI : http://dx.doi.org/10.18462/iir.icr.2023.0533
Liens
Voir d'autres communications du même compte rendu (491)
Voir le compte rendu de la conférence
Indexation
-
An interpretable machine learning method for fa...
- Auteurs : CHEN K., ZHU X., CHEN S., DU Z.
- Date : 21/08/2023
- Langues : Anglais
- Source : Proceedings of the 26th IIR International Congress of Refrigeration: Paris , France, August 21-25, 2023.
- Formats : PDF
Voir la fiche
-
A comprehensive review: Fault detection, diagno...
- Auteurs : SINGH V., MATHUR J., BHATIA A.
- Date : 12/2022
- Langues : Anglais
- Source : International Journal of Refrigeration - Revue Internationale du Froid - vol. 144
- Formats : PDF
Voir la fiche
-
Integration of dynamic model and classification...
- Auteurs : AGUILERA J. J., MEESENBURG W., SCHULTE A., OMMEN T., MARKUSSEN W. B., ZÜHLSDORF B., POULSEN J. L., FÖRSTERLING S., ELMEGAARD B.
- Date : 13/06/2022
- Langues : Anglais
- Source : 15th IIR-Gustav Lorentzen Conference on Natural Refrigerants (GL2022). Proceedings. Trondheim, Norway, June 13-15th 2022.
- Formats : PDF
Voir la fiche
-
An intelligent fault detection and diagnosis mo...
- Auteurs : WANG Z. W., WANG S. C., LI D., CAO Z. W., HE Y. L.
- Date : 04/2024
- Langues : Anglais
- Source : International Journal of Refrigeration - Revue Internationale du Froid - vol. 160
- Formats : PDF
Voir la fiche
-
A semi-supervised data-driven approach for chil...
- Auteurs : FENG Z., WANG L., MA X., JIANG Z., CHANG B.
- Date : 05/04/2023
- Langues : Anglais
- Source : 3rd IIR conference on HFO Refrigerants and low GWP Blends. Shanghai, China.
- Formats : PDF
Voir la fiche