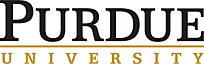
Application de méthodes de classification par apprentissage automatique pour la détection des défaillances et le diagnostic des unités de toiture.
Application of machine learning classification methods in fault detection and diagnosis of rooftop units.
Numéro : 2374
Auteurs : EBRAHIMIFAKHAR A., YUILL D., KABIRIKOPAEI A.
Résumé
In this paper, a data-driven strategy for fault detection and diagnosis in rooftop air conditioning units, based on machine learning classification methods, is proposed. The strategy formulates the fault detection and diagnosis task as a multi-class classification problem. The focus of this study is on detecting and diagnosing the following common rooftop unit faults: refrigerant undercharge, refrigerant overcharge, compressor valve leakage, liquid-line restriction, condenser fouling, evaporator fouling, and non-condensable gas in the refrigerant. Three classification methods, K-nearest neighbors, logistic regression, and random forests were applied to our dataset, and their performance was compared. Ten-fold cross-validation was used to select tuning parameters for different classification methods. Machine learning requires a larger set of training data than could feasibly be generated with experiments, so a library of high-fidelity simulation data was used to train and test the classification methods’ performance. This library contains physical parameters – such as temperatures, pressures, and power consumption – for a three-ton rooftop unit operating with each of the fault types individually, at several fault intensities, over a range of driving conditions. The logistic regression method, with an overall accuracy rate of 93.6%, had the best performance of the methods that were tested. Since class-specific performance is also important in fault detection and diagnosis process, the performance of different classification methods for individual faults was also determined, using true positive rate and false positive rate statistical measures. The results demonstrate the potential of data-driven strategies to detect and diagnose common rooftop unit faults.
Documents disponibles
Format PDF
Pages : 10
Disponible
Prix public
20 €
Prix membre*
15 €
* meilleur tarif applicable selon le type d'adhésion (voir le détail des avantages des adhésions individuelles et collectives)
Détails
- Titre original : Application of machine learning classification methods in fault detection and diagnosis of rooftop units.
- Identifiant de la fiche : 30028469
- Langues : Anglais
- Sujet : Technologie
- Source : 2021 Purdue Conferences. 18th International Refrigeration and Air-Conditioning Conference at Purdue.
- Date d'édition : 05/2021
- Document disponible en consultation à la bibliothèque du siège de l'IIF uniquement.
Liens
Voir d'autres communications du même compte rendu (184)
Voir le compte rendu de la conférence
Indexation
-
Thèmes :
Conditionnement d'air pour le confort;
Circulation du fluide : tuyauterie, régulation, automatisme, sécurité;
Confinement, réduction de la charge en frigorigène - Mots-clés : Apprentissage automatique; Toiture; Conditionnement d'air; Panne; Banque de données; Modélisation; Charge en frigorigène; Fuite
-
Development of a remote refrigerant leakage det...
- Auteurs : KIMURA S., MORIWAKI M., YOSHIMI M., YAMADA S., HIKAWA T., KASAHARA S.
- Date : 10/07/2022
- Langues : Anglais
- Source : 2022 Purdue Conferences. 19th International Refrigeration and Air-Conditioning Conference at Purdue.
- Formats : PDF
Voir la fiche
-
A variable refrigerant flow (VRF) air-condition...
- Auteurs : CHENG H., MU W., CHENG Y., CHEN H., XING L.
- Date : 21/08/2023
- Langues : Anglais
- Source : Proceedings of the 26th IIR International Congress of Refrigeration: Paris , France, August 21-25, 2023.
- Formats : PDF
Voir la fiche
-
A comprehensive review: Fault detection, diagno...
- Auteurs : SINGH V., MATHUR J., BHATIA A.
- Date : 12/2022
- Langues : Anglais
- Source : International Journal of Refrigeration - Revue Internationale du Froid - vol. 144
- Formats : PDF
Voir la fiche
-
A robust fault diagnosis method for HVAC system...
- Auteurs : ZHU X., CHEN S., CHEN K., LIANG X., REN T., JIN X., DU Z.
- Date : 21/08/2023
- Langues : Anglais
- Source : Proceedings of the 26th IIR International Congress of Refrigeration: Paris , France, August 21-25, 2023.
- Formats : PDF
Voir la fiche
-
Methodology to estimate vapor quality of refrig...
- Auteurs : MILLER W., PATEL V., ABU-HEIBA A., et al.
- Date : 24/08/2019
- Langues : Anglais
- Source : Proceedings of the 25th IIR International Congress of Refrigeration: Montréal , Canada, August 24-30, 2019.
- Formats : PDF
Voir la fiche