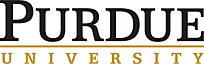
Application of machine learning classification methods in fault detection and diagnosis of rooftop units.
Number: 2374
Author(s) : EBRAHIMIFAKHAR A., YUILL D., KABIRIKOPAEI A.
Summary
In this paper, a data-driven strategy for fault detection and diagnosis in rooftop air conditioning units, based on machine learning classification methods, is proposed. The strategy formulates the fault detection and diagnosis task as a multi-class classification problem. The focus of this study is on detecting and diagnosing the following common rooftop unit faults: refrigerant undercharge, refrigerant overcharge, compressor valve leakage, liquid-line restriction, condenser fouling, evaporator fouling, and non-condensable gas in the refrigerant. Three classification methods, K-nearest neighbors, logistic regression, and random forests were applied to our dataset, and their performance was compared. Ten-fold cross-validation was used to select tuning parameters for different classification methods. Machine learning requires a larger set of training data than could feasibly be generated with experiments, so a library of high-fidelity simulation data was used to train and test the classification methods’ performance. This library contains physical parameters – such as temperatures, pressures, and power consumption – for a three-ton rooftop unit operating with each of the fault types individually, at several fault intensities, over a range of driving conditions. The logistic regression method, with an overall accuracy rate of 93.6%, had the best performance of the methods that were tested. Since class-specific performance is also important in fault detection and diagnosis process, the performance of different classification methods for individual faults was also determined, using true positive rate and false positive rate statistical measures. The results demonstrate the potential of data-driven strategies to detect and diagnose common rooftop unit faults.
Available documents
Format PDF
Pages: 10
Available
Public price
20 €
Member price*
15 €
* Best rate depending on membership category (see the detailed benefits of individual and corporate memberships).
Details
- Original title: Application of machine learning classification methods in fault detection and diagnosis of rooftop units.
- Record ID : 30028469
- Languages: English
- Subject: Technology
- Source: 2021 Purdue Conferences. 18th International Refrigeration and Air-Conditioning Conference at Purdue.
- Publication date: 2021/05
- Document available for consultation in the library of the IIR headquarters only.
Links
See other articles from the proceedings (184)
See the conference proceedings
-
Development of a remote refrigerant leakage det...
- Author(s) : KIMURA S., MORIWAKI M., YOSHIMI M., YAMADA S., HIKAWA T., KASAHARA S.
- Date : 2022/07/10
- Languages : English
- Source: 2022 Purdue Conferences. 19th International Refrigeration and Air-Conditioning Conference at Purdue.
- Formats : PDF
View record
-
A variable refrigerant flow (VRF) air-condition...
- Author(s) : CHENG H., MU W., CHENG Y., CHEN H., XING L.
- Date : 2023/08/21
- Languages : English
- Source: Proceedings of the 26th IIR International Congress of Refrigeration: Paris , France, August 21-25, 2023.
- Formats : PDF
View record
-
An intelligent fault detection and diagnosis mo...
- Author(s) : WANG Z. W., WANG S. C., LI D., CAO Z. W., HE Y. L.
- Date : 2024/04
- Languages : English
- Source: International Journal of Refrigeration - Revue Internationale du Froid - vol. 160
- Formats : PDF
View record
-
A comprehensive review: Fault detection, diagno...
- Author(s) : SINGH V., MATHUR J., BHATIA A.
- Date : 2022/12
- Languages : English
- Source: International Journal of Refrigeration - Revue Internationale du Froid - vol. 144
- Formats : PDF
View record
-
Safe plant rooms for large hydrocarbon chillers.
- Author(s) : GTZ Proklima, TADROS A., MACLAINE-CROSS I., LEONARDI E.
- Date : 2008/07
- Languages : English
View record