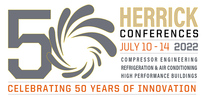
Cadre de classification et de détection des défaillances basé sur la signature électrique pour les pompes à chaleur unitaires à vitesse unique, à l'aide d'une approche de réseau neuronal adaptatif.
Electrical signature based fault classification and detection framework for single-speed, unitary heat pumps using an adaptative neural network approach.
Numéro : 2342
Auteurs : YUILL D., MAMMOLI A., CAUDELL T., GOMATOM P., HU Y., SHOWUNMI O.
Résumé
Automated fault detection and diagnosis (AFDD) for residential air-conditioning systems and heat pumps has significant potential to provide benefits, such as reducing environmental impact, operating cost, energy usage, and peak power required. This is accomplished by addressing faults that would otherwise go unnoticed, or by early detection of progressive faults, such as refrigerant leakage. The key challenge of AFDD development is to provide a method that is both accurate and cost-effective. Since there are very few accessible sensors included in most residential systems, this challenge is often focused on finding a balance between diagnostic accuracy and minimization of sensors.
This paper describes a pilot study to test the hypothesis that high-resolution measurements of electrical power can be used to detect and diagnose faults. An experiment was conducted in which faults were simulated in an air-conditioner under controlled laboratory conditions. The system is a standard 10.5 kW nominal capacity single-speed split system with R-410A refrigerant and a scroll compressor. The faults that were imposed were liquid-line restrictions, improper refrigerant charge, and reduced evaporator airflow. The faults were imposed with a range of typical operating temperatures. Electrical data from startup and from steady operation were gathered in various resolutions, using multiple instrumentation sets capable of sampling frequencies in the 1-20 kHz range. The electrical measurements were made on the outdoor unit, which includes the condenser fan, compressor, and control board. The power signatures of the faulted and fault-free system were compared, using unsupervised machine-learning based methods. The LAPART (laterally primed adaptive resonance theory) approach explores detectable differences between fault and no-fault conditions and classifies voltage and current signatures into learned categories. The initial results show that this method can successfully discriminate between the faulted and fault-free condition at steady state operation, showing that there is potential to develop AFDD methods based upon single-location electrical measurements. Ongoing work will explore the limits of the diagnostic potential from this approach, explore possibilities for simplifying the measurements, and develop practical methods for cloud-based data processing.
Documents disponibles
Format PDF
Pages : 10 p.
Disponible
Gratuit
Détails
- Titre original : Electrical signature based fault classification and detection framework for single-speed, unitary heat pumps using an adaptative neural network approach.
- Identifiant de la fiche : 30030668
- Langues : Anglais
- Sujet : Technologie
- Source : 2022 Purdue Conferences. 19th International Refrigeration and Air-Conditioning Conference at Purdue.
- Date d'édition : 2022
Liens
Voir d'autres communications du même compte rendu (224)
Voir le compte rendu de la conférence
Indexation
-
Thèmes :
Pompes à chaleur à usage domestique;
Conditionnement d'air pour le confort;
Circulation du fluide : tuyauterie, régulation, automatisme, sécurité - Mots-clés : Détection; Fuite; Conditionnement d'air; Secteur résidentiel; Pompe à chaleur domestique; Système split; Réseau neuronal artificiel; Experimentation; Essai; Charge en frigorigène
-
A hybrid deep forest approach for outlier detec...
- Auteurs : ZENG Y., CHEN H., XU C., CHENG Y., GONG Q.
- Date : 12/2020
- Langues : Anglais
- Source : International Journal of Refrigeration - Revue Internationale du Froid - vol. 120
- Formats : PDF
Voir la fiche
-
Evaluation of existing refrigerant charge deter...
- Auteurs : JOUNAY M., CAURET O., TEUILLIERES C., TRAN C. T.
- Date : 21/08/2023
- Langues : Anglais
- Source : Proceedings of the 26th IIR International Congress of Refrigeration: Paris , France, August 21-25, 2023.
- Formats : PDF
Voir la fiche
-
Fault detection and diagnosis of a refrigeratio...
- Auteurs : LIANG Q., HAN H., CUI X., et al.
- Date : 16/08/2015
- Langues : Anglais
- Source : Proceedings of the 24th IIR International Congress of Refrigeration: Yokohama, Japan, August 16-22, 2015.
- Formats : PDF
Voir la fiche
-
A control-oriented hybrid model for a direct ex...
- Auteurs : WANG X., XU X.
- Date : 16/08/2015
- Langues : Anglais
- Source : Proceedings of the 24th IIR International Congress of Refrigeration: Yokohama, Japan, August 16-22, 2015.
- Formats : PDF
Voir la fiche
-
A review on applications of fuzzy logic control...
- Auteurs : BELMAN-FLORES J. M., RODRIGUEZ-VALDERRAMA D. A., LEDESMA S., GARCIA-PABON J. J., HERNÁNDEZ D., PARDO-CELY D. M.
- Date : 02/2022
- Langues : Anglais
- Source : Applied Sciences - vol. 12 - n. 3
- Formats : PDF
Voir la fiche