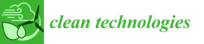
Estimation des économies réalisées par les thermostats Wi-Fi intelligents en matière de contrôle du confort thermique pour tout type de résidence.
Estimating smart Wi-Fi thermostat-enabled thermal comfort control savings for any residence.
Auteurs : ALHAMAYANI A. D., SUN Q., HALLINAN K. P.
Type d'article : Article de périodique
Résumé
Nowadays, most indoor cooling control strategies are based solely on the dry-bulb temperature, which is not close to a guarantee of thermal comfort of occupants. Prior research has shown cooling energy savings from use of a thermal comfort control methodology ranging from 10 to 85%. The present research advances prior research to enable thermal comfort control in residential buildings using a smart Wi-Fi thermostat. “Fanger’s Predicted Mean Vote model” is used to define thermal comfort. A machine learning model leveraging historical smart Wi-Fi thermostat data and outdoor temperature is trained to predict indoor temperature. A Long Short-Term-Memory neural network algorithm is employed for this purpose. The model considers solar heat input estimations to a residence as input features. The results show that this approach yields a substantially improved ability to accurately model and predict indoor temperature. Secondly, it enables a more accurate estimation of potential savings from thermal comfort control. Cooling energy savings ranging from 33 to 47% are estimated based upon real data for variable energy effectiveness and solar exposed residences.
Documents disponibles
Format PDF
Pages : 18
Disponible
Gratuit
Détails
- Titre original : Estimating smart Wi-Fi thermostat-enabled thermal comfort control savings for any residence.
- Identifiant de la fiche : 30029255
- Langues : Anglais
- Sujet : Technologie
- Source : Clean Technologies - vol. 3 - n. 4
- Éditeurs : MDPI
- Date d'édition : 12/2021
- DOI : http://dx.doi.org/10.3390/cleantechnol3040044
Liens
Voir d'autres articles du même numéro (2)
Voir la source
-
Using Deep Learning in Real-Time for Clothing C...
- Auteurs : MEDINA A., MÉNDEZ J. I., PONCE P., PEFFER T., MEIER A., MOLINA A.
- Date : 03/2022
- Langues : Anglais
- Source : Energies - vol. 15 - n. 5
- Formats : PDF
Voir la fiche
-
Informed machine learning to develop a reduced ...
- Auteurs : YOUSAF S., BRADSHAW C. R., KAMALAPURKAR R., SAN O.
- Date : 2022
- Langues : Anglais
- Source : 2022 Purdue Conferences. 19th International Refrigeration and Air-Conditioning Conference at Purdue.
- Formats : PDF
Voir la fiche
-
Recognition of building occupant behaviors from...
- Auteurs : DENG Z.
- Date : 2021
- Langues : Anglais
- Formats : PDF
Voir la fiche
-
Preference-driven personalized thermal control ...
- Auteurs : ZHANG H.
- Date : 12/2023
- Langues : Anglais
- Formats : PDF
Voir la fiche
-
On Hourly Forecasting Heating Energy Consumptio...
- Auteurs : METSÄ-EEROLA I., PULKKINEN J., NIEMITALO O., KOSKELA O.
- Date : 07/2022
- Langues : Anglais
- Source : Energies - vol. 15 - n. 14
- Formats : PDF
Voir la fiche