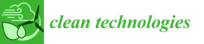
Estimating smart Wi-Fi thermostat-enabled thermal comfort control savings for any residence.
Author(s) : ALHAMAYANI A. D., SUN Q., HALLINAN K. P.
Type of article: Periodical article
Summary
Nowadays, most indoor cooling control strategies are based solely on the dry-bulb temperature, which is not close to a guarantee of thermal comfort of occupants. Prior research has shown cooling energy savings from use of a thermal comfort control methodology ranging from 10 to 85%. The present research advances prior research to enable thermal comfort control in residential buildings using a smart Wi-Fi thermostat. “Fanger’s Predicted Mean Vote model” is used to define thermal comfort. A machine learning model leveraging historical smart Wi-Fi thermostat data and outdoor temperature is trained to predict indoor temperature. A Long Short-Term-Memory neural network algorithm is employed for this purpose. The model considers solar heat input estimations to a residence as input features. The results show that this approach yields a substantially improved ability to accurately model and predict indoor temperature. Secondly, it enables a more accurate estimation of potential savings from thermal comfort control. Cooling energy savings ranging from 33 to 47% are estimated based upon real data for variable energy effectiveness and solar exposed residences.
Available documents
Format PDF
Pages: 18
Available
Free
Details
- Original title: Estimating smart Wi-Fi thermostat-enabled thermal comfort control savings for any residence.
- Record ID : 30029255
- Languages: English
- Subject: Technology
- Source: Clean Technologies - vol. 3 - n. 4
- Publishers: MDPI
- Publication date: 2021/12
- DOI: http://dx.doi.org/10.3390/cleantechnol3040044
Links
See other articles in this issue (2)
See the source
Indexing
-
Using Deep Learning in Real-Time for Clothing C...
- Author(s) : MEDINA A., MÉNDEZ J. I., PONCE P., PEFFER T., MEIER A., MOLINA A.
- Date : 2022/03
- Languages : English
- Source: Energies - vol. 15 - n. 5
- Formats : PDF
View record
-
A neural-network approach to develop algebraic ...
- Author(s) : LIN L., GAO L., HWANG Y., KEDZIERSKI M.
- Date : 2022/07/10
- Languages : English
- Source: 2022 Purdue Conferences. 19th International Refrigeration and Air-Conditioning Conference at Purdue.
- Formats : PDF
View record
-
Energy saving pre-cooling pattern search of an ...
- Author(s) : YOON M. S., YOON W. S.
- Date : 2021/08/31
- Languages : English
- Source: 13th IEA Heat Pump Conference 2021: Heat Pumps – Mission for the Green World. Conference proceedings [full papers]
- Formats : PDF
View record
-
Recognition of building occupant behaviors from...
- Author(s) : DENG Z.
- Date : 2021
- Languages : English
- Formats : PDF
View record
-
Preference-driven personalized thermal control ...
- Author(s) : ZHANG H.
- Date : 2023/12
- Languages : English
- Formats : PDF
View record