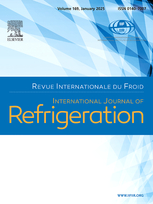
Résumé
Critical temperature () is an important property of refrigerant mixtures. In this study, a neural network-based model is established to predict the of binary mixtures from the molar fraction and molecular structure of their components. First, graph neural network (GNN) is applied to generate the representation of the molecular structure of single component. The generated representation is added according to the molar fraction as the input of the proposed model. In order to embed the prior knowledge, pre-trained parameters are reused in the proposed model to accelerate convergence and improve accuracy. A dataset that contains 66 binary mixtures and 505 data points is collected from reference to adjust the remaining model parameters. The performance of the obtained model is comprehensively evaluated and it shows good prediction accuracy and generalization ability. The average absolute relative deviation (AARD) in the total dataset is 0.80%. For most of the mixtures, the AARD is less than 1%. Finally, the proposed model is compared with three empirical methods and the equation of state (EOS)-based method in REFPROP, and the model in this study show satisfactory result and significant improved accuracy for some mixture types.
Documents disponibles
Format PDF
Pages : 210-220
Disponible
Prix public
20 €
Prix membre*
Gratuit
* meilleur tarif applicable selon le type d'adhésion (voir le détail des avantages des adhésions individuelles et collectives)
Détails
- Titre original : Prediction of critical temperature of binary refrigerant mixtures by neural network.
- Identifiant de la fiche : 30032322
- Langues : Anglais
- Sujet : Technologie
- Source : International Journal of Refrigeration - Revue Internationale du Froid - vol. 161
- Date d'édition : 05/2024
- DOI : http://dx.doi.org/10.1016/j.ijrefrig.2024.02.031
Liens
Voir d'autres articles du même numéro (20)
Voir la source
Indexation
-
Thermodynamic properties of lubricant/refrigera...
- Auteurs : ZOLFAGHARI M., YOUSEFI F.
- Date : 08/2017
- Langues : Anglais
- Source : International Journal of Refrigeration - Revue Internationale du Froid - vol. 80
- Formats : PDF
Voir la fiche
-
Estimation of vapour-liquid equilibria for the ...
- Auteurs : MOHANTY S.
- Date : 03/2006
- Langues : Anglais
- Source : International Journal of Refrigeration - Revue Internationale du Froid - vol. 29 - n. 2
- Formats : PDF
Voir la fiche
-
Prediction of normal boiling point and critical...
- Auteurs : WANG G., HU P.
- Date : 07/2023
- Langues : Anglais
- Source : International Journal of Refrigeration - Revue Internationale du Froid - vol. 151
- Formats : PDF
Voir la fiche
-
Vapor-liquid equilibrium measurement for carbon...
- Auteurs : WU Z., SUN R., SHI L., HU P., TIAN H., WANG X., SHU G.
- Date : 02/2024
- Langues : Anglais
- Source : International Journal of Refrigeration - Revue Internationale du Froid - vol. 158
- Formats : PDF
Voir la fiche
-
Calculation for the thermodynamic properties of...
- Auteurs : SÖZEN A., ÖZALP M., ARCAKLIOGLU E.
- Date : 02/2007
- Langues : Anglais
- Source : Applied Thermal Engineering - vol. 27 - n. 2-3
Voir la fiche