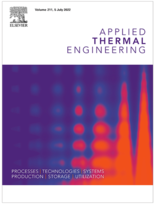
Modèles prédictifs et algorithmes de contrôle pour prédire les chutes de température dans les systèmes de refroidissement.
Prediction models and control algorithms for predictive applications of setback temperature in cooling systems.
Auteurs : MOON J. W., YOON Y., JEON Y., et al.
Type d'article : Article
Résumé
In this study, a temperature control algorithm was developed to apply a setback temperature predictively for the cooling system of a residential building during occupied periods by residents. An artificial neural network (ANN) model was developed to determine the required time for increasing the current indoor temperature to the setback temperature. This study involved three phases: development of the initial ANN-based prediction model, optimization and testing of the initial model, and development and testing of three control algorithms.
The development and performance testing of the model and algorithm were conducted using TRNSYS and MATLAB. Through the development and optimization process, the final ANN model employed indoor temperature and the temperature difference between the current and target setback temperature as two input neurons. The optimal number of hidden layers, number of neurons, learning rate, and moment were determined to be 4, 9, 0.6, and 0.9, respectively. The tangent–sigmoid and pure-linear transfer function was used in the hidden and output neurons, respectively. The ANN model used 100 training data sets with sliding-window method for data management. Levenberg-Marquart training method was employed for model training. The optimized model had a prediction accuracy of 0.9097 root mean square errors when compared with the simulated results.
Employing the ANN model, ANN-based algorithms maintained indoor temperatures better within target ranges. Compared to the conventional algorithm, the ANN-based algorithms reduced the duration of time, in which the indoor temperature was out of the targeted temperature range, as much as 56 and 75 min, respectively. In addition, two ANN-based algorithms removed less heat from indoor space as much as 1.06% and 1.26%. Thus, the applicability of the ANN model and the algorithm presented their potential to be applied for more effective thermal conditioning with reduced energy consumption.
Détails
- Titre original : Prediction models and control algorithms for predictive applications of setback temperature in cooling systems.
- Identifiant de la fiche : 30021071
- Langues : Anglais
- Source : Applied Thermal Engineering - vol. 113
- Date d'édition : 25/02/2017
- DOI : http://dx.doi.org/10.1016/j.applthermaleng.2016.11.087
Liens
Voir d'autres articles du même numéro (24)
Voir la source
Indexation
-
Etude sur les réseaux neuronaux pour un modèle ...
- Auteurs : TANAKA A., KOMINE H., SEKI Y., et al.
- Date : 06/2006
- Langues : Japonais
- Source : Transaction of the Society of Heating, Air-conditioning and Sanitary Engineers of Japan - vol. 111
Voir la fiche
-
Prediction of thermal comfort index predicted m...
- Auteurs : LIU S. B., CAO Q., FU M. X., WANG Y. Y.
- Date : 09/09/1997
- Langues : Anglais
- Source : International Symposium on Air Conditioning in High Rise Buildings - 1997
- Formats : PDF
Voir la fiche
-
Neural model for air exchange in habitable rooms.
- Auteurs : PIOTROWSKI J. Z.
- Date : 09/08/1999
- Langues : Anglais
Voir la fiche
-
ANN-based occupancy detection for energy effici...
- Auteurs : ADHIKARY P., BANDYOPADHYAY S., MAZUMDAR A.
- Date : 27/07/2018
- Langues : Anglais
- Source : Proceedings of the International Conference on Emerging Technologies for Sustainable and Intelligent HVAC&R Systems, Kolkata, July 27-28 2018.
- Formats : PDF
Voir la fiche
-
Prediction and Analysis of Dew Point Indirect E...
- Auteurs : SUN T., HUANG X., LIANG C., LIU R.
- Date : 07/2022
- Langues : Anglais
- Source : Energies - vol. 15 - n. 13
- Formats : PDF
Voir la fiche