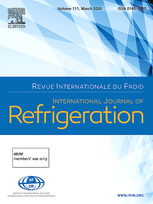
Recommandé par l'IIF / Document IIF
Développement de modèles de réseaux de neurones artificiels à apprentissage approfondi pour prédire la variation de la température et de la demande de puissance pour une application de réponse à la demande dans les entrepôts frigorifiques.
Development of deep learning artificial neural networks models to predict temperature and power demand variation for demand response application in cold storage.
Auteurs : HOANG H. M., AKERMA M., MELLOULI N., LE MONTAGNER A., LEDUCQ D., DELAHAYE A.
Type d'article : Article de la RIF
Résumé
Food warehouses and cold rooms have a significant potential for Demand Response (DR) application (stopping or reducing the power of fans and compressors of the refrigeration system) due to thermal inertia of food products. However, as air and food temperature might increase beyond acceptable limits during DR periods, DR needs to be carefully applied in order to respect the food temperature regulation and to maintain quality and safety of the products. It is thus important to predict the system behaviour in case of DR application in order to evaluate its potential impacts and to decide if DR can be performed or not. Four deep learning artificial neural networks (ANN) models, traditional Long Short-Term Memory LSTM, stacked LSTM, bidirectional LSTM and convolutional LSTM, were developed to predict future temperature and power demand perturbations due to the application of DR in cold storage. The aims of this work are: first, to assess the performance of those models in predicting the system behaviours, in particular the sudden variations during and after DR applications, and second, to identify the impact of data availability (number of sensors, their positions) and data characteristic (quality, quantity and DR patterns) on the prediction performance. The results have shown the high potential of deep learning ANN models in supporting DR application in cold storage.
Documents disponibles
Format PDF
Pages : 857-873
Disponible
Prix public
20 €
Prix membre*
Gratuit
* meilleur tarif applicable selon le type d'adhésion (voir le détail des avantages des adhésions individuelles et collectives)
Détails
- Titre original : Development of deep learning artificial neural networks models to predict temperature and power demand variation for demand response application in cold storage.
- Identifiant de la fiche : 30029190
- Langues : Anglais
- Sujet : Technologie
- Source : International Journal of Refrigeration - Revue Internationale du Froid - vol. 131
- Date d'édition : 11/2021
- DOI : http://dx.doi.org/10.1016/j.ijrefrig.2021.07.029
- Document disponible en consultation à la bibliothèque du siège de l'IIF uniquement.
Liens
Voir d'autres articles du même numéro (95)
Voir la source
-
Prediction of Date Fruit Quality Attributes dur...
- Auteurs : MOHAMMED M., MUNIR M., ALJABR A.
- Date : 06/2022
- Langues : Anglais
- Source : Foods - vol. 11 - n. 11
- Formats : PDF
Voir la fiche
-
Prediction of normal boiling point and critical...
- Auteurs : WANG G., HU P.
- Date : 07/2023
- Langues : Anglais
- Source : International Journal of Refrigeration - Revue Internationale du Froid - vol. 151
- Formats : PDF
Voir la fiche
-
On Hourly Forecasting Heating Energy Consumptio...
- Auteurs : METSÄ-EEROLA I., PULKKINEN J., NIEMITALO O., KOSKELA O.
- Date : 07/2022
- Langues : Anglais
- Source : Energies - vol. 15 - n. 14
- Formats : PDF
Voir la fiche
-
Informed machine learning to develop a reduced ...
- Auteurs : YOUSAF S., BRADSHAW C. R., KAMALAPURKAR R., SAN O.
- Date : 2022
- Langues : Anglais
- Source : 2022 Purdue Conferences. 19th International Refrigeration and Air-Conditioning Conference at Purdue.
- Formats : PDF
Voir la fiche
-
Proposal and Experimental Study on a Diagnosis ...
- Auteurs : LI K., SUN Z., JIN H., XU Y., GU J., HUANG Y., ZHANG Q., SHEN X.
- Date : 03/2022
- Langues : Anglais
- Source : Applied Sciences - vol. 12 - n. 6
- Formats : PDF
Voir la fiche