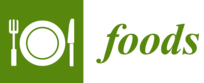
Prédiction des attributs de qualité des dattes pendant le stockage frigorifique en fonction de leurs propriétés électriques à l'aide de modèles de réseaux neuronaux artificiels.
Prediction of Date Fruit Quality Attributes during Cold Storage Based on Their Electrical Properties Using Artificial Neural Networks Models.
Auteurs : MOHAMMED M., MUNIR M., ALJABR A.
Type d'article : Article de périodique
Résumé
Evaluating and predicting date fruit quality during cold storage is critical for ensuring a steady supply of high-quality fruits to meet market demands. The traditional destructive methods take time in the laboratory, and the results are based on one specific parameter being tested. Modern modeling techniques, such as Machine Learning (ML) algorithms, offer unique benefits in nondestructive methods for food safety detection and predicting quality attributes. In addition, the electrical properties of agricultural products provide crucial information about the interior structures of biological tissues and their physicochemical status. Therefore, this study aimed to use an alternative approach to predict physicochemical properties, i.e., the pH, total soluble solids (TSS), water activity (aw), and moisture content (MC) of date fruits (Tamar), during cold storage based on their electrical properties using Artificial Neural Networks (ANNs), which is the most popular ML technique. Ten date fruit cultivars were studied to collect data for the targeted parameters at different cold storage times (0, 2, 4, and 6 months) to train and test the ANNs models. The electrical properties of the date fruits were measured using a high-precision LCR (inductance, capacitance, and resistance) meter from 10 Hz to 100 kHz. The ANNs models were compared with a Multiple Linear Regression (MLR) at all testing frequencies of the electrical properties. The MLR models were less accurate than ANNs models in predicting fruit pH and had low performance and weak predictive ability for the TSS, aw, and MC at all testing frequencies. The optimal ANNs prediction model consisted of the input layer with 14 neurons, one hidden layer with 15 neurons, and the output layer with 4 neurons, which was determined depending on the measurements of the electrical properties at a 10 kHz testing frequency. This optimal ANNs model was able to predict the pH with R2 = 0.938 and RMSE = 0.121, TSS with R2 = 0.954 and RMSE = 2.946, aw with R2 = 0.876 and RMSE = 0.020, and MC with R2 = 0.855 and RMSE = 0.803 b by using the measured electrical properties. The developed ANNs model is a powerful tool for predicting fruit quality attributes after learning from the experimental measurement parameters. It can be suggested to efficiently predict the pH, total soluble solids, water activity, and moisture content of date fruits based on their electrical properties at 10 kHz.
Documents disponibles
Format PDF
Pages : 23 p.
Disponible
Gratuit
Détails
- Titre original : Prediction of Date Fruit Quality Attributes during Cold Storage Based on Their Electrical Properties Using Artificial Neural Networks Models.
- Identifiant de la fiche : 30030382
- Langues : Anglais
- Sujet : Technologie
- Source : Foods - vol. 11 - n. 11
- Éditeurs : MDPI
- Date d'édition : 06/2022
- DOI : http://dx.doi.org/10.3390/foods11111666
Liens
Voir d'autres articles du même numéro (6)
Voir la source
Indexation
-
Development of deep learning artificial neural ...
- Auteurs : HOANG H. M., AKERMA M., MELLOULI N., LE MONTAGNER A., LEDUCQ D., DELAHAYE A.
- Date : 11/2021
- Langues : Anglais
- Source : International Journal of Refrigeration - Revue Internationale du Froid - vol. 131
- Formats : PDF
Voir la fiche
-
Data-driven analysis of risk-assessment methods...
- Auteurs : WANG Q., ZHAO Z., WANG Z.
- Date : 04/2023
- Langues : Anglais
- Source : Foods - vol. 12 - n. 8
- Formats : PDF
Voir la fiche
-
Quality characteristics of bisir "Barhee" dates...
- Auteurs : QURASHI A. D. al-, AWAD M. A.
- Date : 09/2011
- Langues : Anglais
- Source : Fruits - vol. 66 - n. 5
Voir la fiche
-
Smartphone-Based Image Analysis for Rapid Evalu...
- Auteurs : LI H., LV S., FENG L., PENG P., HU L., LIU Z., HATI S., BIMAL C., MO H.
- Date : 07/2022
- Langues : Anglais
- Source : Foods - vol. 11 - n. 14
- Formats : PDF
Voir la fiche
-
Prediction of normal boiling point and critical...
- Auteurs : WANG G., HU P.
- Date : 07/2023
- Langues : Anglais
- Source : International Journal of Refrigeration - Revue Internationale du Froid - vol. 151
- Formats : PDF
Voir la fiche