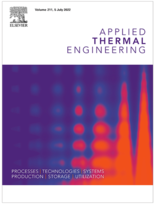
Summary
This paper presents a new fault detection and diagnosis (FDD) method for centrifugal chillers of building air-conditioning systems. Firstly, the Support Vector Regression (SVR) is adopted to develop the reference PI models. A new PI, namely the heat transfer efficiency of the sub-cooling section ( epsilon (sc)), is proposed to improve the FDD performance. Secondly, the Exponentially-Weighted Moving Average (EWMA) control charts are introduced to detect faults in a statistical way to improve the ratios of correctly detected points. Thirdly, when faults are detected, diagnosis follows which is based on a proposed FDD rule table. Six typical chiller component faults are concerned in this paper. This method is validated using the realtime experimental data from the ASHRAE RP-1043. Test results show that the combined use of SVR and EWMA can achieve the best performance. Results also show that significant improvements are achieved compared with a commonly used method using Multiple Linear Regression (MLR) and t-statistic.
Details
- Original title: A statistical fault detection and diagnosis method for centrifugal chillers based on exponentially-weighted moving average control charts and support vector regression.
- Record ID : 30006882
- Languages: English
- Source: Applied Thermal Engineering - vol. 51 - n. 1-2
- Publication date: 2013/03
- DOI: http://dx.doi.org/10.1016/j.applthermaleng.2012.09.030
Links
See other articles in this issue (26)
See the source
Indexing
-
Themes:
Compressors;
Chillers - Keywords: Heat transfer; Chiller; Performance; Failure; Testing; Detection; Centrifugal compressor
-
Decoupling features for fault detection and dia...
- Author(s) : ZHAO X., YANG M., LI H.
- Date : 2011/02
- Languages : English
- Source: HVAC&R Research - vol. 17 - n. 1
View record
-
A simplified physical model-based fault detecti...
- Author(s) : ZHAO Y., WANG S., XIAO F., et al.
- Date : 2013/04
- Languages : English
- Source: HVAC&R Research - vol. 19 - n. 3
View record
-
A fault detection and diagnosis strategy with e...
- Author(s) : XIAO F., ZHENG C. Y., WANG S. W.
- Date : 2011/12
- Languages : English
- Source: Applied Thermal Engineering - vol. 31 - n. 17-18
View record
-
A model-based online fault detection and diagno...
- Author(s) : CUI J., WANG S.
- Date : 2005/10
- Languages : English
- Source: International Journal of thermal Sciences - vol. 44 - n. 10
View record
-
A robust fault detection and diagnosis strategy...
- Author(s) : WANG S., CUI J.
- Date : 2006/07
- Languages : English
- Source: HVAC&R Research - vol. 12 - n. 3
View record