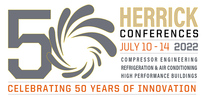
Informed machine learning to develop a reduced order model of unitary equipment.
Number: 2386
Author(s) : YOUSAF S., BRADSHAW C. R., KAMALAPURKAR R., SAN O.
Summary
Combining machine learning tools with conventional reduced order modeling approaches produces the potential for an enormous increase in the ability to select suitable models. This paper presents a machine learning based approach for predicting the cooling capacity of a fixed speed unitary air-conditioner. Experimental data from a 10-ton Roof Top Unit (RTU) is simplified by utilizing a feature selection methodology, Elastic Net (EN), to accurately record and reduce the parameters while simultaneously preserving the physics of the system. The simplified RTU data set resulting from the EN is fed into a novel method for model order reduction employing Principal Component Analysis coupled with a supervised Artificial Neural Network (ANN). Preliminary results show that proposed technique is able to predict the equipment cooling capacity within ±2% of experimental results. Furthermore, the current work has also been compared to the recent models in the literature and has been found to be superior.
Available documents
Format PDF
Pages: 11 p.
Available
Free
Details
- Original title: Informed machine learning to develop a reduced order model of unitary equipment.
- Record ID : 30030706
- Languages: English
- Subject: Technology
- Source: 2022 Purdue Conferences. 19th International Refrigeration and Air-Conditioning Conference at Purdue.
- Publication date: 2022
Links
See other articles from the proceedings (224)
See the conference proceedings
-
Computer simulation of various heat recovery sy...
- Author(s) : LLOPIS-MENGUAL B., ALVAREZ-PINEIRO L., CAZORLA-MARÍN A., NAVARRO-PERIS E.
- Date : 2022/04
- Languages : English
- Source: XI Congreso Ibérico y IX Congreso Iberoamericano de Ciencias y Técnicas del Frío, CYTEF 2022.
- Formats : PDF
View record
-
A high efficiency rooftop air conditioning syst...
- Author(s) : HU Y., SHEN B.
- Date : 2024/11
- Languages : English
- Source: International Journal of Refrigeration - Revue Internationale du Froid - vol. 167
- Formats : PDF
View record
-
Analysis of automated fault detection and diagn...
- Author(s) : EBRAHIMIFAKHAR A., YUILL D., SMITH A. D., GRANDERSON J., CROWE E., CHEN Y., REEVE H.
- Date : 2021/05/24
- Languages : English
- Source: 2021 Purdue Conferences. 6th International High Performance Buildings Conference at Purdue.
- Formats : PDF
View record
-
Field measurement-based validation of fault dia...
- Author(s) : EBRAHIMIFAKHAR A., CHEN Y., YUILL D. P., CROWE E.
- Date : 2022
- Languages : English
- Source: 2022 Purdue Conferences. 19th International Refrigeration and Air-Conditioning Conference at Purdue.
- Formats : PDF
View record
-
Energy saving pre-cooling pattern search of an ...
- Author(s) : YOON M. S., YOON W. S.
- Date : 2021/08/31
- Languages : English
- Source: 13th IEA Heat Pump Conference 2021: Heat Pumps – Mission for the Green World. Conference proceedings [full papers]
- Formats : PDF
View record