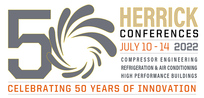
Lagged-kNN based data imputation approach for multi-stream building systems data.
Number: 3182
Author(s) : PRADHAN O., HÄLLENBERG D., CHEN Z., WEN J., WU T., CANDAN K. S., O'NEILL Z.
Summary
Increasing advancements in building digitization, smart sensing, and metering technologies have allowed large amount of data to be collected and saved for monitoring, analyzing, and controlling building systems. However, due to sensors or communications failure, the data collected are often incomplete and poor in quality. Data imputation approaches to replace the missing values, specifically based on both statistical and predictive models have been widely adopted for multivariate datasets. It is hence of interest to find an effective way to impute building system data by leveraging the mutual information from strongly correlated sensors. In this paper, we evaluate multiple data imputation approaches using data collected from a medium sized, mixed-use institution building situated in Stockholm, Sweden. Sensors with widely varying characteristics from the case study building were selected to test the imputation methods. Artificial test data with ground-truth was first created for validation by removing randomly selected portions of data. The imputation accuracy was computed for each method and the impact of the chosen method on a short-term building forecasting model was evaluated. Results demonstrate that incorporating time-lagged cross correlations within the knearest neighbor (kNN) model provide the most accurate imputations.
Available documents
Format PDF
Pages: 10 p.
Available
Free
Details
- Original title: Lagged-kNN based data imputation approach for multi-stream building systems data.
- Record ID : 30030212
- Languages: English
- Source: 2022 Purdue Conferences. 7th International High Performance Buildings Conference at Purdue.
- Publication date: 2022
- Document available for consultation in the library of the IIR headquarters only.
Links
See other articles from the proceedings (39)
See the conference proceedings
Indexing
- Themes: Green buildings
- Keywords: Building; Sensor; Control (automatic); Monitoring; Prediction; Case study; Sweden
-
Régulation par apprentissage : application au c...
- Author(s) : GERASSIMOFF G., JEBALI G., PEFFER T.
- Date : 2018/03
- Languages : French
- Source: Revue générale du Froid & du Conditionnement d'air - n.1168
View record
-
Smart low-cost thermal imaging acquisition towa...
- Author(s) : SOLEIMANIJAVID A., KONSTANTZOS I.
- Date : 2022
- Languages : English
- Source: 2022 Purdue Conferences. 7th International High Performance Buildings Conference at Purdue.
- Formats : PDF
View record
-
Distributed model predictive control for buildi...
- Author(s) : PUTTA V., KIM D., CAI J., et al.
- Date : 2014/07/14
- Languages : English
- Source: 2014 Purdue Conferences. 3rd International High Performance Buildings Conference at Purdue.
- Formats : PDF
View record
-
A tool for generating reduced-order models from...
- Author(s) : KIM D., BAE Y., BRAUN J. E., et al.
- Date : 2018/07/09
- Languages : English
- Source: 2018 Purdue Conferences. 5th International High Performance Buildings Conference at Purdue.
- Formats : PDF
View record
-
Capteurs-actionneurs, face aux exigences de l'a...
- Author(s) : MOREL F.
- Date : 2004/10
- Languages : French
- Source: Process - n. 1208
View record