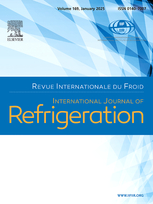
IIR document
Refrigerant leak detection in industrial vapor compression refrigeration systems using machine learning.
Author(s) : MTIBAA A., SESSA V., GUERASSIMOFF G.
Type of article: IJR article
Summary
Efficient detection of refrigerant leakage is of utmost importance for industrial refrigeration systems due to its potential to cause substantial impacts on system performance and the environment. Existing research on fault detection and diagnosis in refrigeration systems primarily revolves around solutions based on experimental or laboratory data. However, in the industrial use case, achieving accurate and early detection poses significant challenges. This paper reports on the development of a novel refrigerant leak detection method for industrial vapor compression refrigeration systems. Our method leverages real-world data obtained from operational installations, enabling us to assess its reliability and applicability. The proposed data-driven approach involves predicting the fault-free liquid level in the installation receiver and comparing the actual and predicted levels. In this work, we place emphasis on features and model selection. Dedicated metrics combined with a model comparison method are proposed to evaluate and compare the performance of commonly used regression models with two sets of features to determine the most effective one. Furthermore, we provide insights into the results obtained from the deployment of the proposed method in real-world industrial installations.
Available documents
Format PDF
Pages: 51-61
Available
Public price
20 €
Member price*
Free
* Best rate depending on membership category (see the detailed benefits of individual and corporate memberships).
Details
- Original title: Refrigerant leak detection in industrial vapor compression refrigeration systems using machine learning.
- Record ID : 30032309
- Languages: English
- Subject: Technology
- Source: International Journal of Refrigeration - Revue Internationale du Froid - vol. 161
- Publication date: 2024/05
- DOI: http://dx.doi.org/10.1016/j.ijrefrig.2024.02.016
Links
See other articles in this issue (20)
See the source
-
An intelligent fault detection and diagnosis mo...
- Author(s) : WANG Z. W., WANG S. C., LI D., CAO Z. W., HE Y. L.
- Date : 2024/04
- Languages : English
- Source: International Journal of Refrigeration - Revue Internationale du Froid - vol. 160
- Formats : PDF
View record
-
A semi-supervised data-driven approach for chil...
- Author(s) : FENG Z., WANG L., MA X., JIANG Z., CHANG B.
- Date : 2023/04/05
- Languages : English
- Source: 3rd IIR conference on HFO Refrigerants and low GWP Blends. Shanghai, China.
- Formats : PDF
View record
-
Integration of dynamic model and classification...
- Author(s) : AGUILERA J. J., MEESENBURG W., SCHULTE A., OMMEN T., MARKUSSEN W. B., ZÜHLSDORF B., POULSEN J. L., FÖRSTERLING S., ELMEGAARD B.
- Date : 2022/06/13
- Languages : English
- Source: 15th IIR-Gustav Lorentzen Conference on Natural Refrigerants (GL2022). Proceedings. Trondheim, Norway, June 13-15th 2022.
- Formats : PDF
View record
-
Optimal subcooling in vapor compression systems...
- Author(s) : KOELN J., ALLEYNE A. G.
- Date : 2014/07
- Languages : English
- Source: International Journal of Refrigeration - Revue Internationale du Froid - vol. 43
- Formats : PDF
View record
-
Empirical modeling and robust control of a nove...
- Author(s) : SUNG T., KIM Y. J., KIM H. S.
- Date : 2017/05
- Languages : English
- Source: International Journal of Refrigeration - Revue Internationale du Froid - vol. 77
- Formats : PDF
View record