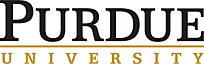
Recommended by the IIR
Reinforcement learning control for vapor compression refrigeration cycle.
Number: 210034
Author(s) : DING T. L., SUBIANTORO A., NORRIS S.
Summary
Vapor compression refrigeration cycles (VCRC) control optimization is an effective method to increase its' reliability and energy efficiency. In modern VCRC systems, the introduction of inverter compressors, electronic expansion valves, fan speed, and pump speed control has significantly increased their controllability. These improvements led to the development of many multivariable and predictive control strategies that improved the system's temperature tracking performance and increased the coefficient of performance (COP) by up to 30% compared to conventional on/off and PID controls. However, a VCRC also has high nonlinearities and parameter couplings, making it difficult to apply these modern control laws. This problem incentivizes the application of reinforcement learning (RL) to optimize VCRC control as RL demonstrated an unprecedented ability to optimize complex control problems. This study explores this idea by using RL to train a direct optimal controller for a VCRC. To test the concept, a VCRC simulation model was developed in the MATLAB Simulink environment to train an RL VCRC controller using the MATLAB reinforcement learning toolbox. The controller's goal is to track the desired internal air temperature and a 10˚C superheat setting. The controller used 17 observations containing the VCRC states, tracking errors, operating conditions, and previous actions to determine the optimal compressor speed and expansion valve opening percentage. The VCRC operating conditions were limited to ambient and internal air temperature ranges of 28-32˚C and 16-20˚C, respectively. This study used the twin delayed deep deterministic policy gradient (TD3) RL algorithm to train the controller. The TD3 training hyperparameters such as the noise model and deep neural network parameters were tuned to balance the exploration and exploitation of the solution space. The training converged to a suboptimal solution after completing 6500 episodes in 5 days using an Intel Core i7-8700 CPU 3.2GHz with 32 GB RAM. The developed RL controller was tested using custom ambient and internal air temperature profiles. The controller tracked both the internal air and superheat temperature settings well with low error and fast response time. However, when the ambient temperature fell below 29˚C, the actuators began to fluctuate, indicating that it did not learn a good policy for this region. This study showed that RL could optimize VCRC control, but more research is necessary to improve it.
Available documents
Format PDF
Pages: 10
Available
Public price
20 €
Member price*
15 €
* Best rate depending on membership category (see the detailed benefits of individual and corporate memberships).
Details
- Original title: Reinforcement learning control for vapor compression refrigeration cycle.
- Record ID : 30028605
- Languages: English
- Subject: Technology
- Source: 2021 Purdue Conferences. 18th International Refrigeration and Air-Conditioning Conference at Purdue.
- Publication date: 2021/05
- Document available for consultation in the library of the IIR headquarters only.
Links
See other articles from the proceedings (184)
See the conference proceedings
Indexing
- Themes: Compression systems
- Keywords: Optimization; Simulation; Thermodynamic property; Control (generic); Compression system; Modelling
-
Application of mixed integer nonlinear programm...
- Author(s) : BRENDEL L. P. M., BRAUN J. E., GROLL E. A.
- Date : 2022
- Languages : English
- Source: 2022 Purdue Conferences. 19th International Refrigeration and Air-Conditioning Conference at Purdue.
- Formats : PDF
View record
-
Model predictive control of vapor compression s...
- Author(s) : JAIN N., BURNS D. J., DI CAIRANO S., et al.
- Date : 2014/07/14
- Languages : English
- Source: 2014 Purdue Conferences. 15th International Refrigeration and Air-Conditioning Conference at Purdue.
- Formats : PDF
View record
-
On the difficulty of globally optimally control...
- Author(s) : BEJARANO G., ALFAYA J. A., ORTEGA M. G., et al.
- Date : 2017/01/25
- Languages : English
- Source: Applied Thermal Engineering - vol. 111
View record
-
Development of a fast method for retrieving the...
- Author(s) : MA J., KIM D., BRAUN J. E.
- Date : 2018/07/09
- Languages : English
- Source: 2018 Purdue Conferences. 17th International Refrigeration and Air-Conditioning Conference at Purdue.
- Formats : PDF
View record
-
Numerical study and experimental validation of ...
- Author(s) : RIGOLA J., PÉREZ-SEGARRA C. D., GARCÍA-VALLADARES O., SERRA J. M., ESCRIBÀ M., PONS J.
- Date : 1998/07/14
- Languages : English
- Source: Proceedings of the 1998 Purdue International Refrigeration Conference.
View record