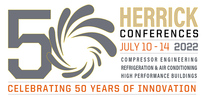
Recommended by the IIR
Using machine learning for feature selection in automated fault detection and diagnosis of split system air conditioners.
Number: 2351
Author(s) : CHEN Y., EBRAHIMIFAKHAR A., YUILL D.
Summary
Because of the advancement of smart buildings and smart sensors, enriched data with respect to building operation is now available, making the data-driven approach an appealing method for fault detection and diagnosis in air-conditioners. Data-driven methods, unlike many other fault diagnosis methods, necessitate a substantial quantity of data to develop a model, which limits their deployment in the field. Another issue with using such an approach is the variables' availability. From a cost-effectiveness standpoint, the input variables need to be chosen very carefully, since they constitute a large portion of the cost of applying fault diagnostics. This study provides two feature selection strategies for use in machine learning-based diagnostic techniques, using real-world practical considerations in combination with the SVM classifier, to investigate how the reduction of features affects the fault diagnosis performance of the data-driven approach. This study used a high-fidelity data set with a total of 15 variables, in which seven faults were simulated under various driving conditions. The full data set was split into a training set, with 70% of the original data, and a test set, accounting for 30%. During the training process, the 10-fold cross-validation method was employed to tune the parameters of the model. Compared to the balanced accuracy of 98.9% when all features were used, the removal of the features by sequential backward selection process produced 97.2% balanced accuracy which was only slightly lower. In terms of the feature selection based on real-world availability, 94.7% of balanced accuracy was obtained, indicating that this method can still achieve acceptable accuracy. Precision, recall, and F1 scores are also provided in the paper, to further describe the diagnostic performance for each fault in the data.
Available documents
Format PDF
Pages: 10 p.
Available
Free
Details
- Original title: Using machine learning for feature selection in automated fault detection and diagnosis of split system air conditioners.
- Record ID : 30030687
- Languages: English
- Subject: Technology
- Source: 2022 Purdue Conferences. 19th International Refrigeration and Air-Conditioning Conference at Purdue.
- Publication date: 2022
Links
See other articles from the proceedings (224)
See the conference proceedings
Indexing
- Themes: Other air-conditioning equipment
- Keywords: Machine learning; Detection; Split system; Air conditioning; Simulation; Modelling; Default
-
A robust fault diagnosis method for HVAC system...
- Author(s) : ZHU X., CHEN S., CHEN K., LIANG X., REN T., JIN X., DU Z.
- Date : 2023/08/21
- Languages : English
- Source: Proceedings of the 26th IIR International Congress of Refrigeration: Paris , France, August 21-25, 2023.
- Formats : PDF
View record
-
A prediction method for overall economic value ...
- Author(s) : YUILL D. P., BRAUN J. E.
- Date : 2016/07/11
- Languages : English
- Source: 2016 Purdue Conferences. 16th International Refrigeration and Air-Conditioning Conference at Purdue.
- Formats : PDF
View record
-
Semi-supervised diagnosis method of refrigerati...
- Author(s) : LI K., JIN H., XU Y., GU J., HUANG Y., SHI L., YAO Q., SHEN X.
- Date : 2024/02
- Languages : English
- Source: International Journal of Refrigeration - Revue Internationale du Froid - vol. 158
- Formats : PDF
View record
-
Integration of dynamic model and classification...
- Author(s) : AGUILERA J. J., MEESENBURG W., SCHULTE A., OMMEN T., MARKUSSEN W. B., ZÜHLSDORF B., POULSEN J. L., FÖRSTERLING S., ELMEGAARD B.
- Date : 2022/06/13
- Languages : English
- Source: 15th IIR-Gustav Lorentzen Conference on Natural Refrigerants (GL2022). Proceedings. Trondheim, Norway, June 13-15th 2022.
- Formats : PDF
View record
-
Research on fault diagnosis strategy of air-con...
- Author(s) : MA Q., YUE C., YU M., SONG Y., CUI P., YU Y.
- Date : 2024/02
- Languages : English
- Source: International Journal of Refrigeration - Revue Internationale du Froid - vol. 158
- Formats : PDF
View record