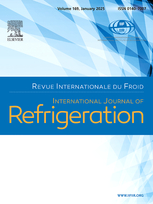
Summary
The utilization of electronic expansion valves (EEVs) in refrigeration and air conditioning systems is increased for energy saving and comfort environmental. However, experimental data and refrigerant mass flow models through EEVs are very limited in open literature. In this study, a new technique using artificial neural network (ANN) is applied to depict the mass flow rates of R22 and its alternatives R407C and R410A flowing through EEVs based on the error back propagation learning algorithm. Two strategies are followed; the first is to construct individual ANN models for each refrigerant, and the second is to construct a generalized ANN model for the three investigated refrigerants. The experimental results from open literature are used to construct the ANN models. The ANN models results showed a good agreement with the corresponding experimental data. For individual models, the relative deviations for R22, R407C, and R410A are within ±0.7%, ±1.1%, and ±0.006%, respectively. While for generalized model, the relative deviations are within ±2.5%. Also the generalized model was tested out of its construction range in a predictive mode and it was found to be a reliable tool to estimate the refrigerants mass flow rates.
Available documents
Format PDF
Pages: 113-124
Available
Public price
20 €
Member price*
Free
* Best rate depending on membership category (see the detailed benefits of individual and corporate memberships).
Details
- Original title: Artificial neural network models for depicting mass flow rate of R22, R407C and R410A through electronic expansion valves.
- Record ID : 30016731
- Languages: English
- Source: International Journal of Refrigeration - Revue Internationale du Froid - vol. 63
- Publication date: 2016/03
Links
See other articles in this issue (19)
See the source
Indexing
-
Themes:
HCFCs;
HFCs;
Expansion systems - Keywords: Refrigerating system; R410A; R407C; Electronic expansion valve; Artificial neural network; R22
-
Experimental research on the refrigerant mass f...
- Author(s) : MA S., ZHANG C., CHEN J., et al.
- Date : 2005/10
- Languages : English
- Source: Applied Thermal Engineering - vol. 25 - n. 14-15
View record
-
Refrigerant flow through electronic expansion v...
- Author(s) : CAO X., LI Z. Y., SHAO L. L., et al.
- Date : 2016/01/05
- Languages : English
- Source: Applied Thermal Engineering - vol. 92
View record
-
Generalized neural network correlation for flow...
- Author(s) : WANG W. J., ZHAO L. X., ZHANG C. L.
- Date : 2006/07
- Languages : English
- Source: International Journal of Heat and Mass Transfer - vol. 49 - n. 15-16
View record
-
An electronic expansion valve modeling framewor...
- Author(s) : WAN H., CAO T., HWANG Y., et al.
- Date : 2019/11
- Languages : English
- Source: International Journal of Refrigeration - Revue Internationale du Froid - vol. 107
- Formats : PDF
View record
-
Electronic expansion valve mass flow rate predi...
- Author(s) : TIAN Z., GU B., QIAN C., et al.
- Date : 2015/09
- Languages : English
- Source: International Journal of Refrigeration - Revue Internationale du Froid - vol. 57
- Formats : PDF
View record