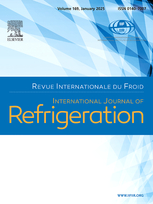
IIR document
Evaporating temperature estimation of refrigeration systems based on vibration data-driven soft sensors.
Author(s) : SEIXAS BUSSE DE SIQUEIRA NASCIMENTO A., ZOMER MACHADO J. P., DOS SANTOS COELHO L., COSTA FLESCH R. C.
Type of article: IJR article
Summary
The evaluation of the operating conditions of refrigeration compressors once installed in household appliances is challenging due to the need to install pressure transducers, a process which requires system evacuation and refrigerant reintroduction. In addition, changes in the piping modify the characteristics of the original product. This paper proposes a soft-sensing technique based on vibration measurements of the compressor surface to predict the evaporating temperature. Different machine learning (ML) techniques are evaluated as data-driven prediction models, namely multilayer perceptron (MLP) neural networks, least squares boosting, generalized additive model, random forest, extreme learning machine, and random vector functional link neural networks. These techniques were applied to data obtained from a test rig designed to emulate compressor operation in a refrigeration system, with an operating envelope from -30 °C to -10 °C for the evaporating temperature and from 34 °C to 54 °C for the condensing temperature. The results showed that, with a single vibration measurement point, it was possible to use an MLP technique to estimate the evaporating temperature with a root mean squared error of 1.74 °C in a non-intrusive way. For the other prediction techniques, the errors were a bit higher than for the MLP, but the maximum error value was about 2.5 °C in all cases.
Available documents
Format PDF
Pages: 288-296
Available
Public price
20 €
Member price*
Free
* Best rate depending on membership category (see the detailed benefits of individual and corporate memberships).
Details
- Original title: Evaporating temperature estimation of refrigeration systems based on vibration data-driven soft sensors.
- Record ID : 30032860
- Languages: English
- Source: International Journal of Refrigeration - Revue Internationale du Froid - vol. 168
- Publication date: 2024/12
- DOI: http://dx.doi.org/10.1016/j.ijrefrig.2024.08.020
Links
See other articles in this issue (63)
See the source
Indexing
- Themes: Compressors
- Keywords: Compressor; Refrigerant; Machine learning; Artificial neural network; Test rig; Expérimentation
-
Proposal and Experimental Study on a Diagnosis ...
- Author(s) : LI K., SUN Z., JIN H., XU Y., GU J., HUANG Y., ZHANG Q., SHEN X.
- Date : 2022/03
- Languages : English
- Source: Applied Sciences - vol. 12 - n. 6
- Formats : PDF
View record
-
Training neural networks to predict the energy ...
- Author(s) : PATIL S., PONNUSAMI S., KOVACEVIC A., ASATI N.
- Date : 2022/07/15
- Languages : English
- Source: 2022 Purdue Conferences. 26th International Compressor Engineering Conference at Purdue.
- Formats : PDF
View record
-
Parallel deep neural network for scalable coupl...
- Author(s) : CHEN S., LIU Z., CHEN K., ZHU X., JIN X., DU Z.
- Date : 2023/08/21
- Languages : English
- Source: Proceedings of the 26th IIR International Congress of Refrigeration: Paris , France, August 21-25, 2023.
- Formats : PDF
View record
-
Development of deep learning artificial neural ...
- Author(s) : HOANG H. M., AKERMA M., MELLOULI N., LE MONTAGNER A., LEDUCQ D., DELAHAYE A.
- Date : 2021/11
- Languages : English
- Source: International Journal of Refrigeration - Revue Internationale du Froid - vol. 131
- Formats : PDF
View record
-
Prediction of Date Fruit Quality Attributes dur...
- Author(s) : MOHAMMED M., MUNIR M., ALJABR A.
- Date : 2022/06
- Languages : English
- Source: Foods - vol. 11 - n. 11
- Formats : PDF
View record