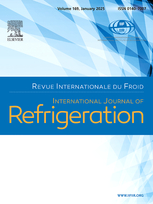
IIR document
Forecasting operation of a chiller plant facility using data-driven models.
Author(s) : SALIMIAN RIZI B., FARAMARZI A., PERTZBORN A., HEIDARINEJAD M.
Type of article: IJR article
Summary
In recent years, data-driven models have enabled accurate prediction of chiller power consumption and chiller coefficient of performance (COP). This study evaluates the usage of time series Extreme Gradient Boosting (XGBoost) models to predict chiller power consumption and chiller COP of a water-cooled chiller plant. The 10-second measured data used in this study are from the Intelligent Building Agents Laboratory (IBAL), which includes two water-cooled chillers. Preprocessing, data selection, noise analysis, and data smoothing methods influence the accuracy of these data-driven predictions. The data intervals were changed to 30 s, 60 s, and 180 s using down-sampling and averaging strategies to investigate the impact of data preprocessing methods and data resolutions on the accuracy of chiller COP and power consumption models. To overcome the effect of noise on the accuracy of the models of chiller power consumption and COP, two data smoothing methods, the moving average window strategy and the Savitzky-Golay (SG) filter, are applied. The results show that both methods improve the predictions compared to the baseline, with the SG filter slightly outperforming the moving average. Particularly, the mean absolute percentage error of the chiller COP and power consumption models improved from 4.8 to 4.9 for the baseline to 1.9 and 2.3 with the SG filter, respectively. Overall, this study provides a practical guide to developing XGBoost data-driven chiller power consumption and COP prediction models.
Available documents
Format PDF
Pages: 70-89
Available
Public price
20 €
Member price*
Free
* Best rate depending on membership category (see the detailed benefits of individual and corporate memberships).
Details
- Original title: Forecasting operation of a chiller plant facility using data-driven models.
- Record ID : 30032792
- Languages: English
- Source: International Journal of Refrigeration - Revue Internationale du Froid - vol. 167
- Publication date: 2024/11
- DOI: http://dx.doi.org/10.1016/j.ijrefrig.2024.07.019
Links
See other articles in this issue (22)
See the source
Indexing
-
Themes:
Chillers;
Energy efficiency, energy savings - Keywords: Chiller; COP; Energy consumption; Prediction; Model
-
Attention mechanism-aided model ensemble method...
- Author(s) : CAI J., YANG H.
- Date : 2024/09
- Languages : English
- Source: International Journal of Refrigeration - Revue Internationale du Froid - vol. 165
- Formats : PDF
View record
-
Prediction of chiller power consumption using t...
- Author(s) : FAN C., XIAO F., WANG S.
- Date : 2013/06/16
- Languages : English
- Source: Clima 2013. 11th REHVA World Congress and 8th International Conference on Indoor Air Quality, Ventilation and Energy Conservation in Buildings.
- Formats : PDF
View record
-
Optimal chiller loading by improved parallel pa...
- Author(s) : GAO Z., YU J., HU Q., YANG S.
- Date : 2022/04
- Languages : English
- Source: International Journal of Refrigeration - Revue Internationale du Froid - vol. 136
- Formats : PDF
View record
-
Energiebesparende schroefcompressor-technologie.
- Author(s) : MÖLLER A., NEUS M.
- Date : 2011/03
- Languages : Dutch
- Source: Koude & Luchtbehandeling - vol. 104
View record
-
Optimising energy use in refrigeration systems.
- Author(s) : PEARSON A.
- Date : 2021/09/16
- Languages : English
- Source: 9th IIR Conference on Ammonia and CO2 Refrigeration Technologies. Proceedings: Ohrid, North Macedonia, 16-17 September, 2021
- Formats : PDF
View record