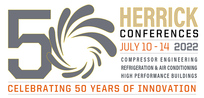
Summary
The gray-box modeling approach (i.e., semi-physical thermal network model) has been widely used for prediction applications for buildings such as a model predictive control (MPC). However, applying the modeling approach for practical buildings is still challenging due to unmeasured disturbances such as occupants, lighting, appliances, and in/exfiltration loads. To overcome this problem, several system identification approaches have been proposed by considering the dynamics of unmeasured disturbance. However, the performance of long-term (e.g., one day) zone temperature or load predictions could still be very poor, and this is an important research topic for enabling grid-interactive buildings. In this study, we propose a hybrid modeling approach to improve long-term temperature or load predictions. Several system identification approaches for gray-box models are compared using simulations to understand the limitations. A neural network model that accounts for unmeasured disturbance is developed by considering the limitation of the graybox model and is combined with the gray-box model. This hybrid model approach shows 0.24°C root mean squared error (RMSE) for 1-day ahead temperature prediction on average, while the conventional gray-box model shows 1.1°C RMSE on average.
Available documents
Format PDF
Pages: 10 p.
Available
Free
Details
- Original title: Hybrid modeling approach for better identification of building thermal network model and improved prediction.
- Record ID : 30030244
- Languages: English
- Source: 2022 Purdue Conferences. 7th International High Performance Buildings Conference at Purdue.
- Publication date: 2022
- Document available for consultation in the library of the IIR headquarters only.
Links
See other articles from the proceedings (39)
See the conference proceedings
-
Optimal load shifting for multiple ON/OFF air c...
- Author(s) : KIM D., BRAUN J. E.
- Date : 2022
- Languages : English
- Source: 2022 Purdue Conferences. 7th International High Performance Buildings Conference at Purdue.
- Formats : PDF
View record
-
Inherent capacity modulation of a linear refrig...
- Author(s) : ZHU Z., LIANG K., CHEN H., MENG Z.
- Date : 2022/11
- Languages : English
- Source: International Journal of Refrigeration - Revue Internationale du Froid - vol. 143
- Formats : PDF
View record
-
A distributed approach to efficient model predi...
- Author(s) : PUTTA V., ZHU G., KIM D., et al.
- Date : 2012/07/16
- Languages : English
- Source: 2012 Purdue Conferences. 2nd International High Performance Buildings Conference at Purdue.
- Formats : PDF
View record
-
Model-based predictive control for buildings wi...
- Author(s) : KIM D., BRAUN J. E.
- Date : 2012/07/16
- Languages : English
- Source: 2012 Purdue Conferences. 2nd International High Performance Buildings Conference at Purdue.
- Formats : PDF
View record
-
Laboratory assessment of a demand response cont...
- Author(s) : SARHADIAN R., COBURN B. A., MENDOZA A., et al.
- Date : 2018/07/09
- Languages : English
- Source: 2018 Purdue Conferences. 17th International Refrigeration and Air-Conditioning Conference at Purdue.
- Formats : PDF
View record