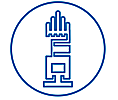
IIR document
Machine learning and high-throughput screening algorithms for optimization of magnetocaloric effect in all-d-metal Heusler alloys.
Number: 0026
Author(s) : BAIGUTLIN D., SOKOLOVSKIY V., BUCHELNIKOV V., TASKAEV S.
Summary
This paper examines the application of regression models using active machine learning techniques to predict the structural and magnetic properties, as well as to estimate the magnetocaloric effect of all-d metal Heusler alloys. The accuracy of the model was determined by cross-validation using the coefficient of determination R2 and the root mean square error RMSE. The model predictions were compared with experimental data and the results of density functional theory (DFT) calculations. The resulting regression model exhibits high accuracy for structural properties, although difficulties in predicting magnetic moments are noted due to the limited representation of magnetic states in the training dataset. The model is capable of qualitatively predicting martensitic transition stoppages for Ni-Co(Fe)-Mn-Ti systems. An improvement of the model could be achieved by extending the training data set to include other possible magnetic states and types of structural disorder.
Available documents
Format PDF
Pages: 6
Available
Public price
20 €
Member price*
Free
* Best rate depending on membership category (see the detailed benefits of individual and corporate memberships).
Details
- Original title: Machine learning and high-throughput screening algorithms for optimization of magnetocaloric effect in all-d-metal Heusler alloys.
- Record ID : 30032634
- Languages: English
- Subject: Technology
- Source: 10th IIR Conference on Caloric Cooling and Applications of Caloric Materials
- Publication date: 2024/08/24
- DOI: http://dx.doi.org/10.18462/iir.thermag.2024.0026
Links
See other articles from the proceedings (29)
See the conference proceedings
-
Optimization of active magnetic regenerators wi...
- Author(s) : CARARO J. E., LOZANO J. A., TREVIZOLI P. V., et al.
- Date : 2016/09/11
- Languages : English
- Source: 7th International Conference on Magnetic Refrigeration at Room Temperature (Thermag VII). Proceedings: Turin, Italy, September 11-14, 2016.
- Formats : PDF
View record
-
How to build the optimal magnet assembly for ma...
- Author(s) : WIESHEU M., MERKEL M., SITTIG T., BENKE D., FRIES M., SCHÖPS S., WEEGER O., CORTES GARCIA I.
- Date : 2023/08
- Languages : English
- Source: International Journal of Refrigeration - Revue Internationale du Froid - vol. 152
- Formats : PDF
View record
-
Magnetocaloric effect: theoretical description ...
- Author(s) : OLIVEIRA N. A. de, RANKE P. J. von, TROPER A.
- Date : 2012/09/17
- Languages : English
- Source: 5th International Conference on Magnetic Refrigeration at Room Temperature (Thermag V). Proceedings: Grenoble, France, September 17-20, 2012.
- Formats : PDF
View record
-
Peculiarities of the magnetocaloric effect in t...
- Author(s) : GIMAEV R. R., ZVEREV V. I., SALETSKY A. M., et al.
- Date : 2016/09/11
- Languages : English
- Source: 7th International Conference on Magnetic Refrigeration at Room Temperature (Thermag VII). Proceedings: Turin, Italy, September 11-14, 2016.
- Formats : PDF
View record
-
A novel magnetic valve using room temperature m...
- Author(s) : ERIKSEN D., BAHL C. R. H., PRYDS N., et al.
- Date : 2012/09/17
- Languages : English
- Source: 5th International Conference on Magnetic Refrigeration at Room Temperature (Thermag V). Proceedings: Grenoble, France, September 17-20, 2012.
- Formats : PDF
View record