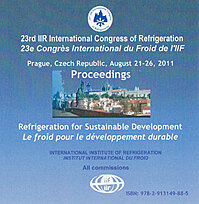
IIR document
Prediction of flow boiling heat transfer in helically coiled tubes using neural network.
Number: pap. ID: 849
Author(s) : ELSAYED A., DADAH R. K. al-, MAHMOUD S., et al.
Summary
Flow boiling through helical coils is an effective heat transfer enhancement technique where the centripetal force distributes the liquid film on the wall resulting in thinner liquid film and higher critical heat flux. Although there are several empirical correlations in the literature, most of these correlations are applicable for specific operating conditions. Recently, Artificial Neural Networks (ANNs) technique has been used for performance prediction in various thermal engineering topics. This paper presents the application of feed forward neural network with Levenberg-Marquardt training algorithm to predict the heat transfer coefficients of flow boiling inside 2.8mm diameter helically coiled tube. The Normalized Prandtl, Dean, Convective and Boiling numbers were utilized as network input while the two-phase to liquid only heat transfer coefficients ratio was used as output. This network was trained using 353 data points from published literature and validated with data from current experimental measurements with deviation of ± 30%.
Available documents
Format PDF
Pages: 8 p.
Available
Public price
20 €
Member price*
Free
* Best rate depending on membership category (see the detailed benefits of individual and corporate memberships).
Details
- Original title: Prediction of flow boiling heat transfer in helically coiled tubes using neural network.
- Record ID : 30001839
- Languages: English
- Source: Proceedings of the 23rd IIR International Congress of Refrigeration: Prague, Czech Republic, August 21-26, 2011. Overarching theme: Refrigeration for Sustainable Development.
- Publication date: 2011/08/21
Links
See other articles from the proceedings (569)
See the conference proceedings
Indexing
-
Model for prediction of flow boiling heat trans...
- Author(s) : LI M., DANG C., TANAKA S., et al.
- Date : 2010/06/07
- Languages : English
- Source: ACRA2010. Asian conference on refrigeration and air conditioning: Tokyo, Japan, June 7-9, 2010.
- Formats : PDF
View record
-
Development of general correlation for heat tra...
- Author(s) : INOUE N., GOTO M.
- Date : 2010/06/07
- Languages : English
- Source: ACRA2010. Asian conference on refrigeration and air conditioning: Tokyo, Japan, June 7-9, 2010.
- Formats : PDF
View record
-
A new model for flow boiling heat transfer coef...
- Author(s) : KANIZAWA F. T., MOGAJI T. S., RIBATSKI G.
- Date : 2016/01
- Languages : English
- Source: International Journal of Refrigeration - Revue Internationale du Froid - vol. 61
- Formats : PDF
View record
-
Correlation for the flow boiling heat transfer ...
- Author(s) : MIYATA K., MORI H., HAMAMOTO Y.
- Date : 2011/08/21
- Languages : English
- Source: Proceedings of the 23rd IIR International Congress of Refrigeration: Prague, Czech Republic, August 21-26, 2011. Overarching theme: Refrigeration for Sustainable Development.
- Formats : PDF
View record
-
Dry-out CHF correlation for R134a flow boiling ...
- Author(s) : CHEN C. N., HAN J. T., JEN T. C., et al.
- Date : 2011/01
- Languages : English
- Source: International Journal of Heat and Mass Transfer - vol. 54 - n. 1-3
View record