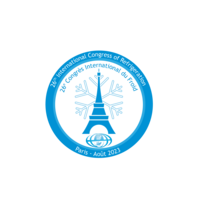
Summary
This paper proposes a stacking ensemble learning method to detect the refrigerant charge fault of the variable refrigerant flow (VRF) air conditioning system. Firstly, measurement data is collected from the refrigerant charge fault experiment of a VRF system performed in an enthalpy difference laboratory. Several features are selected based on different criteria after preprocessing the measurement data and importance analysis. To prevent overfitting, no duplicate dataset is created. Then, five classification models have been used in this paper as follows: Support Vector Machine (SVM), Gradient Boosting Machine (GBM), Random Forest (RF), Multi-layer Perceptron (MLP), and K-Nearest Neighbors (KNN). After several parameter optimizations, these models demonstrate accuracy between 84.71% to 91.58%. Finally, different stacking ensemble learning methods integrate these models, achieving the test set's best accuracy rate (96.66%).
Available documents
Format PDF
Pages: 14
Available
Public price
20 €
Member price*
Free
* Best rate depending on membership category (see the detailed benefits of individual and corporate memberships).
Details
- Original title: A variable refrigerant flow (VRF) air-conditioning system refrigerant charge detection method using stacking ensemble learning.
- Record ID : 30031850
- Languages: English
- Subject: Technology
- Source: Proceedings of the 26th IIR International Congress of Refrigeration: Paris , France, August 21-25, 2023.
- Publication date: 2023/08/21
- DOI: http://dx.doi.org/10.18462/iir.icr.2023.0936
Links
See other articles from the proceedings (491)
See the conference proceedings
Indexing
-
Development of a remote refrigerant leakage det...
- Author(s) : KIMURA S., MORIWAKI M., YOSHIMI M., YAMADA S., HIKAWA T., KASAHARA S.
- Date : 2022/07/10
- Languages : English
- Source: 2022 Purdue Conferences. 19th International Refrigeration and Air-Conditioning Conference at Purdue.
- Formats : PDF
View record
-
Application of machine learning classification ...
- Author(s) : EBRAHIMIFAKHAR A., YUILL D., KABIRIKOPAEI A.
- Date : 2021/05
- Languages : English
- Source: 2021 Purdue Conferences. 18th International Refrigeration and Air-Conditioning Conference at Purdue.
- Formats : PDF
View record
-
Research on fault diagnosis strategy of air-con...
- Author(s) : MA Q., YUE C., YU M., SONG Y., CUI P., YU Y.
- Date : 2024/02
- Languages : English
- Source: International Journal of Refrigeration - Revue Internationale du Froid - vol. 158
- Formats : PDF
View record
-
Quantitative detection of refrigerant charge fa...
- Author(s) : ZHAO T., YANG J., ZHU J., PENG M., LU C., SHI Z.
- Date : 2025/01
- Languages : English
- Source: International Journal of Refrigeration - Revue Internationale du Froid - vol. 169
- Formats : PDF
View record
-
Deep learning-based refrigerant charge fault de...
- Author(s) : EOM Y. H., HONG S. B., YOO J. W., KIM M. S.
- Date : 2021/08/31
- Languages : English
- Source: 13th IEA Heat Pump Conference 2021: Heat Pumps – Mission for the Green World. Conference proceedings [full papers]
- Formats : PDF
View record