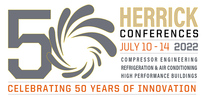
Adaptive reinforcement learning PI controllers for vapor compression cycle control.
Number: 2165
Author(s) : DING T. L., NORRIS S., SUBIANTORO A.
Summary
Reinforcement Learning (RL) showed high potential to learn optimal control policies for Vapor Compression Cycles (VCC). However, RL lacks robustness outside the range of training data, increasing the risk of directly applying RL for VCC control. Therefore, two adaptive RL-PI controllers were developed for VCC control to merge the benefits of RL and the stability of PI controllers. Each adaptive RL-PI controller has an RL agent that adapts the feedback gains of two PI controllers regulating the compressor speed and expansion valve opening. The controllers must track chamber temperature (CT) and degree of superheat (SH) setpoints in varying cooling loads, ambient temperatures, and fan speeds. Both controllers were trained using the Soft Actor-Critic algorithm, but they used different reward functions that either prioritized the CT or SH. The controllers were trained using a simulated Air Conditioner (AC). During testing, both RL-PI controllers successfully regulated the CT and SH reliably. Both controllers achieved lower SH mean absolute error (MAE) than a dual-PI controller with static gains. However, they obtained higher CT MAE as they sacrificed CT accuracy to reduce the refrigerant mass flow rate and SH fluctuations. The effect of the reward function was also highlighted in the controller’s response, where the RL-PI CT controller had higher CT accuracy but lower SH accuracy than the RL-PI SH controller. A sensitivity analysis also confirmed this finding where the RL-PI CT controller showed a high bias towards CT observations. Finally, the sensitivity analysis also showed that both RL-PI controllers adapted gains considering both actuators, the VCC operating conditions, and the tracking performance.
Available documents
Format PDF
Pages: 10 p.
Available
Free
Details
- Original title: Adaptive reinforcement learning PI controllers for vapor compression cycle control.
- Record ID : 30030483
- Languages: English
- Subject: Technology
- Source: 2022 Purdue Conferences. 19th International Refrigeration and Air-Conditioning Conference at Purdue.
- Publication date: 2022/07/10
Links
See other articles from the proceedings (224)
See the conference proceedings
Indexing
-
Refrigerant leak detection in industrial vapor ...
- Author(s) : MTIBAA A., SESSA V., GUERASSIMOFF G.
- Date : 2024/05
- Languages : English
- Source: International Journal of Refrigeration - Revue Internationale du Froid - vol. 161
- Formats : PDF
View record
-
Experimental study on a cascade chiller for air...
- Author(s) : PALOMBA V., ROSA D. La, VASTA S., et al.
- Date : 2018/06/18
- Languages : English
- Source: 13th IIR Gustav Lorentzen Conference on Natural Refrigerants (GL2018). Proceedings. Valencia, Spain, June 18-20th 2018.
- Formats : PDF
View record
-
A platform-based product family design method u...
- Author(s) : KOBAYASHI T., HAMADA S., NAKAGAWA N., IKEDA H., HATTORI H., KODAMA T., ZHOU X., LU Y., NAGAI H.
- Date : 2022
- Languages : English
- Source: 2022 Purdue Conferences. 19th International Refrigeration and Air-Conditioning Conference at Purdue.
- Formats : PDF
View record
-
Thermodynamic design and simulation of a CO2 ba...
- Author(s) : AHAMMED E., BHATTACHARYYA S., RAMGOPAL M.
- Date : 2014/09
- Languages : English
- Source: International Journal of Refrigeration - Revue Internationale du Froid - vol. 45
- Formats : PDF
View record
-
Machine-learning-based compressor models: A cas...
- Author(s) : WAN H., CAO T., HWANG Y., CHANG S. D., YOON Y. J.
- Date : 2021/03
- Languages : English
- Source: International Journal of Refrigeration - Revue Internationale du Froid - vol. 123
- Formats : PDF
View record