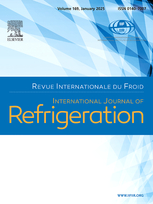
Recommended by the IIR / IIR document
Machine-learning-based compressor models: A case study for variable refrigerant flow systems.
Author(s) : WAN H., CAO T., HWANG Y., CHANG S. D., YOON Y. J.
Type of article: IJR article
Summary
Conventionally, researchers conducted field tests and modeling works for the Variable Refrigerant Flow system separately. In this study, we used the compressor model as a case study to illustrate a novel approach to integrate field tests and modeling works. Field tests in an office building were conducted to collect data. For mass flow rate prediction, three traditional models, including the 20-coefficient model, the efficiency-based model, and the efficiency-based 20-coefficient model, and three machine-learning-based models including Support Vector Regression, Neural Network, and Random Forest, were investigated and compared. We found that the efficiency-based 20-coefficient model and the Support Vector Regression model had a higher accuracy (the mean relative errors were 0.11% and 0.15%, and the coefficient of variation of the root mean-square-error were 0.20 and 0.23) and lower uncertainty within 0.2 g•s−1 for steady-state operation prediction. However, all six models failed to predict accurately the beginning part of the transient process. A tens-of-seconds delay existed between the predicted values and the experiment values. We applied the Convolutional-Neural-Network-based model to address this problem. The mean relative error of this model is reduced to 2% for dynamic simulation. In summary, we recommend the efficiency-based 20-coefficient model, and the Support Vector Regression model for the steady-state compressor model development, while the Convolutional-Neural-Network-based model is recommended for transient model development. For the power consumption prediction, 20-coefficient and Neural Network models can predict transient data well. The process of predicting the capacity is the same as the process of mass flow rate prediction.
Available documents
Format PDF
Pages: 23-33
Available
Public price
20 €
Member price*
Free
* Best rate depending on membership category (see the detailed benefits of individual and corporate memberships).
Details
- Original title: Machine-learning-based compressor models: A case study for variable refrigerant flow systems.
- Record ID : 30028150
- Languages: English
- Subject: Technology
- Source: International Journal of Refrigeration - Revue Internationale du Froid - vol. 123
- Publication date: 2021/03
- DOI: http://dx.doi.org/10.1016/j.ijrefrig.2020.12.003
- Document available for consultation in the library of the IIR headquarters only.
Links
See other articles in this issue (19)
See the source
Indexing
-
Preference-driven personalized thermal control ...
- Author(s) : ZHANG H.
- Date : 2023/12
- Languages : English
- Formats : PDF
View record
-
Development of dynamic modeling framework using...
- Author(s) : WAN H., CAO T., HWANG Y., CHIN S.
- Date : 2021/05
- Languages : English
- Source: 2021 Purdue Conferences. 18th International Refrigeration and Air-Conditioning Conference at Purdue.
- Formats : PDF
View record
-
On Hourly Forecasting Heating Energy Consumptio...
- Author(s) : METSÄ-EEROLA I., PULKKINEN J., NIEMITALO O., KOSKELA O.
- Date : 2022/07
- Languages : English
- Source: Energies - vol. 15 - n. 14
- Formats : PDF
View record
-
Recognition of building occupant behaviors from...
- Author(s) : DENG Z.
- Date : 2021
- Languages : English
- Formats : PDF
View record
-
Evaporating temperature estimation of refrigera...
- Author(s) : SEIXAS BUSSE DE SIQUEIRA NASCIMENTO A., ZOMER MACHADO J. P., DOS SANTOS COELHO L., COSTA FLESCH R. C.
- Date : 2024/12
- Languages : English
- Source: International Journal of Refrigeration - Revue Internationale du Froid - vol. 168
- Formats : PDF
View record