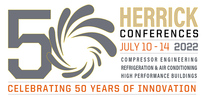
Summary
Ejectors have found its application in improving the performance of carbon dioxide refrigeration systems over the last three decades. As the cycle complexity increases with the addition of an ejector, it is imperative to design the ejector cycle after thorough system analysis. The system model requires accurate heat exchanger models, and therefore, detailed Finite Volume (FV) models are needed. Though the FV heat exchanger models are accurate, they can be computationally expensive which hampers an extensive component analysis during the design phase. In this study, a computationally efficient Artificial Neural Network (ANN) evaporator model is developed from the wide range of experimental data of a given evaporator, operated in a standard ejector cycle. Usually, several number of temporal data points are collected for a single steady state data point. Though, the steady state data has less uncertainty than the temporal data, it is difficult and time consuming to collect large number of steady-state performance data points. This limits the number of neurons in the ANN, as the model run into an overfitting problem. In this study, the ANN evaporator model is developed using both the steady state (331 data points) and the temporal data (28,853 data points). The ANN model is developed after optimizing the neural network topology while using different activation functions and backpropagation techniques. The study finds that the ANN based on temporal data does not show any improvement over ANN based on steady state data. The ANN model with 2 layers (4 neurons in the first layer, and 16 neurons in the second layer) is found to have the minimum residual. The ANN model is trained using Bayesian Regularization backpropagation technique with symmetric sigmoid as the activation function. The ANN model predicts 99.7% of the data points, whereas the FV model with 100 elements, predicts 91.2% of the data points within ± 10% accuracy for the capacity when compared with the experimental data. The ANN model takes around 15milliseconds, whereas FV model with 10 elements, takes around 12-19 seconds to compute evaporator performance. Later, both the ANN and the FV evaporator models are used in a standard ejector cycle system model. The system model with FV (100 elements) predicts 79% of the data points, whereas the system with ANN evaporator model predicts 100% of the data points within ±10% accuracy. The system using ANN model is found to be 139 times faster than the FV (10 elements) based system model. This shows that the developed ANN model can be useful in conducting an extensive component selection analysis as it is not only computationally fast, but also accurate. The applicability of the technique extends to other refrigerant systems as well.
Available documents
Format PDF
Pages: 10 p.
Available
Free
Details
- Original title: Developing computationally efficient artificial neural network model of R744 microchannel evaporator from experimental data for component selection analysis of an ejector system.
- Record ID : 30030710
- Languages: English
- Subject: Technology
- Source: 2022 Purdue Conferences. 19th International Refrigeration and Air-Conditioning Conference at Purdue.
- Publication date: 2022
Links
See other articles from the proceedings (224)
See the conference proceedings
-
Prediction of two-phase flow distribution in mi...
- Author(s) : GIANNETTI N., REDO M. A., SHOLAHUDIN S., JEONG J., YAMAGUCHI S., SAITO K., KIM H.
- Date : 2020/03
- Languages : English
- Source: International Journal of Refrigeration - Revue Internationale du Froid - vol. 111
- Formats : PDF
View record
-
Experimental investigation of an ultrahigh-lift...
- Author(s) : HERDEN D., XU Y., BARTA R. B., THOMAS C.
- Date : 2024/08
- Languages : English
- Source: 16th IIR-Gustav Lorentzen Conference on Natural Refrigerants (GL2024). Proceedings. University of Maryland, College Park, Maryland, USA, August 11-14 2024
- Formats : PDF
View record
-
Thermal modeling of gas engine driven air to wa...
- Author(s) : SANAYE S., ASGARI H.
- Date : 2013/12
- Languages : English
- Source: International Journal of Refrigeration - Revue Internationale du Froid - vol. 36 - n. 8
- Formats : PDF
View record
-
Performance simulation and diagnosis of faulty ...
- Author(s) : LI Y., HU H., LEI R.
- Date : 2023/12
- Languages : English
- Source: International Journal of Refrigeration - Revue Internationale du Froid - Vol. 156
- Formats : PDF
View record
-
Using artificial neural network for predicting ...
- Author(s) : KORKMAZ M. E., GÜMÜSEL L., MARKAL B.
- Date : 2012/09
- Languages : English
- Source: International Journal of Refrigeration - Revue Internationale du Froid - vol. 35 - n. 6
- Formats : PDF
View record