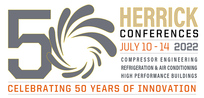
Recursive bayesian calibration of data-driven archetype building energy models for residential sector: application to a research house.
Number: 3465
Author(s) : ABTAHI S. M., ATHIENITIS A. K., DELCROIX B.
Summary
This paper studies recursive Bayesian calibration of archetype building energy models developed for optimal operation, energy flexibility and resilience in the residential sector. Real-time building performance monitoring has been facilitated recently with low cost faster instrumentations. With an online stream of measured data, recursive model calibration can be employed, where the model is initialized with prior knowledge and is updated with new measurements available to the building automation system. A Markov chain Monte Carlo approach is applied to calibrate two multi-zone archetype models with data from a research house in Québec. Most likely parameter values are estimated with an iterative Metropolis-Hastings algorithm over a 5-day train period and are validated by comparing the measured indoor air temperature in each zone with the model output over another 5-day test period. The time series of the posterior probability distribution shows that heat source activation influences the maximum likelihood values of most parameters and remarkably narrows down their associated credible intervals. Analyzing probability distribution time series helps understand the progression of acquired knowledge with new data and facilitates the comparison of various models.
Available documents
Format PDF
Pages: 10 p.
Available
Free
Details
- Original title: Recursive bayesian calibration of data-driven archetype building energy models for residential sector: application to a research house.
- Record ID : 30030236
- Languages: English
- Source: 2022 Purdue Conferences. 7th International High Performance Buildings Conference at Purdue.
- Publication date: 2022
Links
See other articles from the proceedings (39)
See the conference proceedings
Indexing
- Themes: Green buildings
- Keywords: Energy; Energy consumption; Modelling; Building; Case study; Canada
-
Harnessing climate.
- Author(s) : KUWABARA B., AUER T., AKERSTREAM T., et al.
- Date : 2011
- Languages : English
- Source: High Performing Buildings - Fall 2011
View record
-
Reducing energy use in older large buildings.
- Author(s) : ARNOLD D.
- Date : 2017/03
- Languages : English
- Source: EcoLibrium - vol. 17 - n. 2
- Formats : PDF
View record
-
Dedicated to efficiency. 2016 ASHRAE Technology...
- Author(s) : LEMIRE N., FREDERICKS R. O., BARIL P. L., et al.
- Date : 2016/10
- Languages : English
- Source: EcoLibrium - vol. 15 - n. 9
- Formats : PDF
View record
-
A heuristic model predictive control method to ...
- Author(s) : MOROVAT N., ATHIENITIS A. K., CANDANEDO J. A.
- Date : 2022
- Languages : English
- Source: 2022 Purdue Conferences. 7th International High Performance Buildings Conference at Purdue.
- Formats : PDF
View record
-
Energy analysis of a 3D printed building and en...
- Author(s) : REVERTE V., MASIP X., PRADES-GIL C., BARCELÓ RUESCAS F.
- Date : 2020/11/11
- Languages : English
- Source: X Congreso Ibérico y VIII Congreso Iberoamericano de Ciencias y Técnicas del Frío, CYTEF 2020.
- Formats : PDF
View record