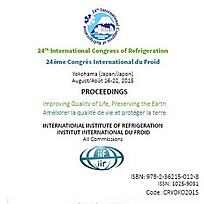
Summary
This paper presents a new Response Surface Methodology based neural network approach to model the refrigerant flow through adiabatic capillary tube. Experimental data of ten different refrigerants in the literatures covering subcooled, two-phase and supercritical inlet conditions are collected as the database, which plays as an experiment rig. Box-Behnken design (BBD) and Central Composite design (CCD) are applied to determine a small dataset for neural network training. With BBD, 25 sets of data are selected for neural network training and the average deviation (A.D.), standard deviation (S.D.) and coefficient of determination (R2) of trained neural network for all data are 2.6%, 9.6% and 0.948, respectively. With CCD, 22 sets of data are selected and the A.D., S.D. and R2 for all data are 0.05%, 10.2% and 0.934, respectively. In addition, the results show that the proposed model is superior than classical polynomial response surface model in such a nonlinear problem.
Available documents
Format PDF
Pages: 8 p.
Available
Public price
20 €
Member price*
Free
* Best rate depending on membership category (see the detailed benefits of individual and corporate memberships).
Details
- Original title: Modeling of refrigerant flow through adiabatic capillary tubes using neural network and response surface methodology.
- Record ID : 30015268
- Languages: English
- Source: Proceedings of the 24th IIR International Congress of Refrigeration: Yokohama, Japan, August 16-22, 2015.
- Publication date: 2015/08/16
- DOI: http://dx.doi.org/10.18462/iir.icr.2015.0107
Links
See other articles from the proceedings (657)
See the conference proceedings
Indexing
-
Themes:
HFCs;
Expansion systems;
Ammonia;
CO2;
Blends - Keywords: Capillary tube; Artificial neural network; Modelling; Flow; Refrigerant
-
Generalized correlation of refrigerant mass flo...
- Author(s) : KIM H. J.
- Date : 2005/06
- Languages : English
- Source: International Journal of Refrigeration - Revue Internationale du Froid - vol. 28 - n. 4
- Formats : PDF
View record
-
Assessment of existing dimensionless correlatio...
- Author(s) : SHAO L. L., WANG J. C., JIN X. C., et al.
- Date : 2013/01
- Languages : English
- Source: International Journal of Refrigeration - Revue Internationale du Froid - vol. 36 - n. 1
- Formats : PDF
View record
-
Model-based neural network correlation for refr...
- Author(s) : ZHANG C. L., ZHAO L. X.
- Date : 2007/06
- Languages : English
- Source: International Journal of Refrigeration - Revue Internationale du Froid - vol. 30 - n. 4
- Formats : PDF
View record
-
Performance prediction of adiabatic capillary t...
- Author(s) : KALEEM KHAN M., KUMAR R., SAHOO P. K.
- Date : 2009/01/25
- Languages : English
- Source: ASHRAE Transactions. Papers presented at the 2009 ASHRAE Winter Conference: Chicago, Illinois, January 2009. Volume 115, part 1.
View record
-
Modified neural network correlation of refriger...
- Author(s) : YANG L., ZHANG C. L.
- Date : 2009/09
- Languages : English
- Source: International Journal of Refrigeration - Revue Internationale du Froid - vol. 32 - n. 6
- Formats : PDF
View record