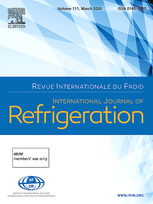
Recommended by the IIR / IIR document
Prediction of flammability classifications of refrigerants by artificial neural network and random forest model.
Author(s) : DEVOTTA S., CHELANI A., VONSILD A.
Type of article: IJR article
Summary
The flammability classification of refrigerants, as per existing standards, is bounded by multiple boundaries and involves many error bands and uncertainties. Further, experimental determination involves significant efforts and costs. Therefore, as a preamble to any refrigerant development efforts, it is desirable to have an assessment method, to predict a priori, the flammability class. In this work, the flammability classifications are predicted by both Artificial Neural Network and Random Forest models using the composition of the refrigerant molecules as predictors, including number of C, H, F, Cl, Br, I, O and N atoms and number of double bonds. Additionally, HR, a ratio between the total atomic mass of H atoms to the molecule's molar mass, is also used to account for the fact that a molecule's flammability increases with increase in number of H atom in the molecule. Both ANN and RF models predict the flammability classifications of totally 179 refrigerants (47 single components and 132 blends) accurately except for three and one refrigerant blend respectively. The level of certainty by the proposed models is considered to be acceptable for a preliminary assessment.
Available documents
Format PDF
Pages: 947-955
Available
Public price
20 €
Member price*
Free
* Best rate depending on membership category (see the detailed benefits of individual and corporate memberships).
Details
- Original title: Prediction of flammability classifications of refrigerants by artificial neural network and random forest model.
- Record ID : 30029197
- Languages: English
- Subject: Regulation, HFCs alternatives
- Source: International Journal of Refrigeration - Revue Internationale du Froid - vol. 131
- Publication date: 2021/11
- DOI: http://dx.doi.org/10.1016/j.ijrefrig.2021.07.021
- Document available for consultation in the library of the IIR headquarters only.
Links
See other articles in this issue (95)
See the source
-
Fault detection and diagnosis of a refrigeratio...
- Author(s) : LIANG Q., HAN H., CUI X., et al.
- Date : 2015/08/16
- Languages : English
- Source: Proceedings of the 24th IIR International Congress of Refrigeration: Yokohama, Japan, August 16-22, 2015.
- Formats : PDF
View record
-
Generalized correlation of refrigerant mass flo...
- Author(s) : KIM H. J.
- Date : 2005/06
- Languages : English
- Source: International Journal of Refrigeration - Revue Internationale du Froid - vol. 28 - n. 4
- Formats : PDF
View record
-
An overview of how flammable refrigerants are c...
- Author(s) : SPLETZER S., COUGHLAN P., GERSTEL J.
- Date : 2018/09
- Languages : English
- Source: 1st IIR international conference on the application of HFO refrigerants, Birmingham, September 2-5 2018.
- Formats : PDF
View record
-
An overview of how flammable refrigerants are c...
- Author(s) : SPLETZER S., COUGHLAN P., GERSTEL J.
- Date : 2018/09
- Languages : English
- Source: 1st IIR international conference on the application of HFO refrigerants, Birmingham, September 2-5 2018.
- Formats : PDF
View record
-
Prediction of global warming potentials of refr...
- Author(s) : DEVOTTA S., CHELANI A., VONSILD A.
- Date : 2021/11
- Languages : English
- Source: International Journal of Refrigeration - Revue Internationale du Froid - vol. 131
- Formats : PDF
View record